How RAG Improves Customer Service Efficiency and Accuracy
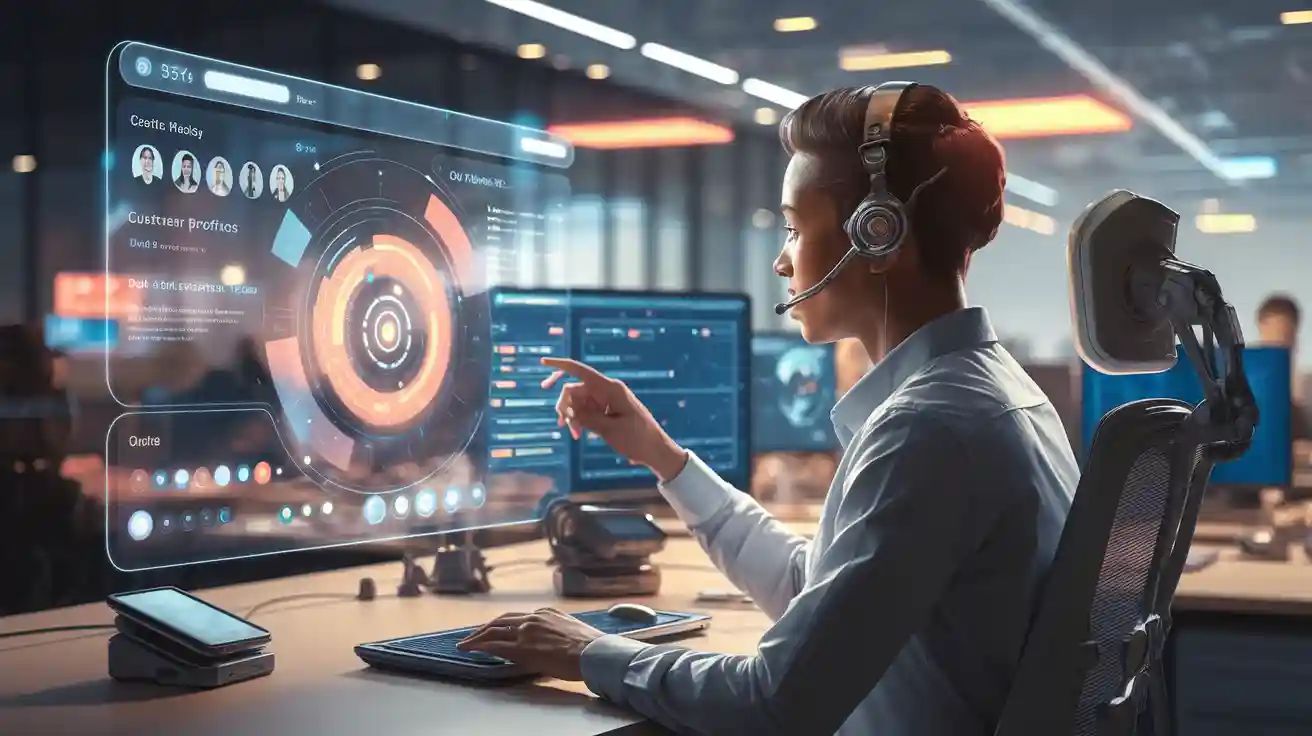
Businesses today face increasing demands for faster and more accurate customer service. RAG-based customer service addresses this challenge by combining real-time data retrieval with AI-driven text generation. It enables systems to provide precise answers while maintaining context, ensuring a seamless customer experience. Unlike traditional methods, rag based customer service reduces errors and enhances efficiency by delivering relevant responses tailored to individual inquiries. This innovation transforms customer support, allowing organizations to handle complex queries with speed and accuracy, ultimately improving customer satisfaction and loyalty.
Key Takeaways
- RAG technology uses live data and AI to give correct answers fast.
- Using RAG can make response times shorter, making customers happier.
- RAG systems are more accurate and make fewer mistakes, building trust.
- RAG helps businesses give custom answers using customer info, boosting loyalty.
- Adding RAG can simplify work, save money, and make businesses stand out.
Understanding RAG in Customer Support
What Is Retrieval-Augmented Generation?
Retrieval-Augmented Generation (RAG) is an advanced AI framework that combines real-time data retrieval with natural language generation. It retrieves relevant information from external databases or documents and uses this data to generate accurate and contextually appropriate responses. Unlike traditional AI models that rely solely on pre-trained knowledge, RAG dynamically integrates up-to-date information, ensuring its outputs remain relevant and precise.
The technical foundation of RAG lies in its ability to process large datasets efficiently. Key performance metrics such asaccuracy, precision, and recall at k (Recall@k) highlight its effectiveness. For instance, accuracy measures the proportion of correct responses, while metrics like MAP@K and MRR@K evaluate retrieval quality. These capabilities make RAG a powerful tool for automating customer interactions and enhancing customer service automation.
Metric | Description |
---|---|
Accuracy | Proportion of true results (both true positives and true negatives) among total cases examined. |
Precision | Fraction of relevant instances among retrieved instances (TP/(TP+FP)). |
Recall at k (Recall@k) | Fraction of relevant instances retrieved over total relevant cases, considering top k results. |
MAP@K | Mean Average Precision at K, a common metric for evaluating retrieval effectiveness. |
MRR@K | Mean Reciprocal Rank at K, another metric for assessing retrieval quality. |
Why RAG Is Essential for Modern Customer Service
Modern customer service demands speed, accuracy, and adaptability. RAG addresses these needs by enabling systems to retrieve real-time, verified data and generate responses tailored to individual queries. This capability ensures that customer support interactions are both efficient and accurate. Businesses leveragingRAG-powered solutions can reduce response times, improve support ticket accuracy, and enhance customer satisfaction.
Thegrowing adoption of RAG in sectors like e-commerce and healthcare underscores its importance. Organizations increasingly rely on RAG to manage complex inquiries and deliver personalized experiences. By automating customer interactions, RAG not only improves operational efficiency but also ensures that responses reflect the latest industry trends and regulatory changes.
Evidence Description | Impact on Operational Metrics |
---|---|
RAG enhances efficiency, accuracy, and security across business processes. | Improves overall operational efficiency and accuracy. |
By integrating RAG-powered real-time insights, businesses can enhance customer interactions, improve support ticket accuracy, and reduce response time. | Reduces response times and improves accuracy in customer support. |
RAG pulls from real-time, verified sources to generate responses that reflect the latest industry data, regulatory changes, and market trends. | Ensures responses are accurate and up-to-date, enhancing decision-making. |
Limitations of Traditional Customer Support Approaches
Traditional customer support systems often struggle with limitations in autonomy, context management, and adaptability. These systems rely on predefined processes, making them less effective in dynamic or complex scenarios. For example, they may fail to manage long-term context or infer user intent accurately, leading to suboptimal customer experiences.
In contrast, RAG-based methods excel in these areas. They adjust responses based on context, manage user intent effectively, and adapt to changing environments. This adaptability makes RAG a superior choice for customer support automation, particularly in industries requiring high levels of precision and personalization.
Aspect | Traditional Customer Support | RAG-based Methods |
---|---|---|
Autonomy | Limited autonomy, follows predefined processes | Enhanced autonomy, adjusts based on context |
Context Management | Struggles with long-term context and user intent | Manages context effectively, infers user intent |
Adaptability | Works well in static environments | Excels in dynamic, complex scenarios |
Mechanisms of RAG for Improved Efficiency and Accuracy
Real-Time Data Retrieval for Accurate Responses
RAG leverages real-time data retrieval to ensure accurate customer support responses. This mechanism involves dynamically pulling relevant information from external sources, such as databases, documents, or APIs, and integrating it into the response generation process. By doing so, RAG systems provide context-rich responses that reflect the most up-to-date information available.
The process begins withdocument loading, where various types of files, including PDFs, Word documents, and spreadsheets, are imported using tools like LangChain Document Loaders. These documents are then segmented into smaller, manageable pieces to facilitate efficient processing. Next, embeddings are generated using models like OpenAI's GPT-4, which transform document segments into vector representations for comparison. The system stores these embeddings in a vector database, enabling hybrid retrieval methods to identify the most relevant segments based on user queries. Finally, GPT-4 combines the retrieved data with the query to generate accurate and contextually appropriate responses.
Key Insight: Research highlights that fine-tuning RAG systems significantly enhances response accuracy, even when irrelevant or conflicting data is present. This ensures that customer interactions remain reliable and precise.Real-World Impact:
- RAG-powered systems have improved search accuracy by 35% in applications like search engines.
- Businesses using real-time data retrieval report a30% increase in engagement rates and a 25% boost in customer satisfaction.
Contextual Relevance Through Ranking and Filtering
To deliver context-rich responses, RAG employs advancedranking and filtering techniques. These methods prioritize the most relevant information by analyzing user intent and interaction history.Neural re-ranking models, for instance, outperform traditional keyword-based systems by capturing listwise context and adapting to user-specific needs.
The ranking process often combines dense and sparse retrieval methods, creating hybrid models that optimize both speed and precision. Context-aware techniques further enhance personalization by adjusting rankings based on previous interactions. For example, customer support chatbots using BERT-based re-rankers have achieved a 25% increase in resolution rates by prioritizing relevant guides over generic FAQs.
Evidence Type | Description |
---|---|
Neural Re-ranking Models | Capture listwise context and user intent, outperforming traditional keyword-based systems in relevance. |
Context-aware Techniques | Adapt rankings based on user interaction history, enhancing personalization and response timing. |
Hybrid Retrieval Models | Combine dense and sparse methods for improved speed and precision, optimizing performance in high-traffic systems. |
AI-Driven Generation of Personalized Interactions
RAG's ability to generate personalized interactions stems from its integration of AI-driven natural language generation. By analyzing customer data, such as past interactions, preferences, and sentiments, RAG systems craft responses that feel human-like and empathetic. This personalization not only improves customer satisfaction but also strengthens brand loyalty.
Machine learning models enable RAG systems to tailor responses dynamically. For instance, AI tools can suggest products based on individual customer data, enhancing marketing and upselling efforts. Additionally, sentiment analysis allows chatbots to adjust their tone, ensuring that responses resonate with the customer's emotional state.
Benefits of RAG-Based Customer Service
Faster Response Times and Reduced Wait Times
RAG-based customer service significantly reduces response times by leveraging real-time data retrieval and AI-driven processing. Traditional customer service systems often rely on manual processes or outdated databases, which can delay responses. In contrast, RAG systems dynamically pull real-time information from external sources, ensuring that customers receive accurate answers almost instantly.
For example, a RAG-powered chatbot can access a company's knowledge base, retrieve relevant data, and generate a response within seconds. This capability eliminates the need for customers to wait for human agents to research and respond. Businesses using RAG systems report faster decision-making and improved operational efficiency, as these systems handle multiple queries simultaneously without compromising quality.
Pro Tip: Companies can enhance customer satisfaction by integrating RAG systems into their support platforms, ensuring 24/7 availability and reduced wait times.Enhanced Accuracy and Reduced Errors
RAG systems excel in delivering accurate and error-free responses by combiningreal-time information retrieval with advanced AI algorithms. Unlike traditional systems, which may rely on outdated or incomplete data, RAG ensures that responses are both contextually relevant and up-to-date. This accuracy enhances the overall customer experience and builds trust in the service.
Research highlights several improvements achieved through RAG systems:
- A28% increase in technical terminology accuracy.
- A 35% improvement in context preservation during translations.
- A 40% boost in cultural adaptation accuracy.
- A 45% higher BLEU score in translation tasks compared to traditional systems.
- A 40% reduction in translation errors.
These metrics demonstrate how RAG enhances customer interactions by minimizing errors and ensuring precise communication. For instance, a multinational bank that implemented RAG technology reported a30% increase in customer satisfaction due to improved response accuracy.
Metric | Description |
---|---|
User Satisfaction | Measures success through end-user satisfaction surveys and engagement metrics. |
Update Responsiveness | Assesses how quickly the system incorporates new information into responses. |
Case Study Example | A multinational bank saw a 30% increase in customer satisfaction after RAG implementation. |
Scalable Solutions for High-Volume Customer Support
RAG-based systems provide scalable solutions for handling high volumes of customer interactions. Traditional customer service models often struggle to manage peak periods due to limited human resources. RAG systems, however, utilize large language models (LLMs) and advanced hardware to process multiple queries simultaneously, ensuring consistent performance even during high-traffic periods.
Key features that enable scalability include:
Feature | Description |
---|---|
Content Generation via LLMs | Utilizes large language models to generate contextually relevant responses to customer queries. |
Multi-Modal Input Processing | Processes both voice and text inputs for comprehensive customer interaction handling. |
Knowledge Base Integration Module | Ingests and retrieves data from customer support databases, ensuring access to up-to-date information. |
High-Performance Hardware Setup | Runs on powerful hardware for low-latency and scalable operations, meeting real-time processing needs. |
Intel Gaudi 3 Accelerators | Supports handling of large language models and concurrent requests, enabling high throughput. |
Improved Customer Satisfaction Through Personalization
Personalization has become a cornerstone of modern customer service, and RAG-based customer service excels in delivering tailored experiences. By leveraging real-time data retrieval and AI-driven generation, RAG systems analyze customer preferences, past interactions, and contextual information to craft responses that resonate on a personal level. This approach not only enhances customer satisfaction but also fosters loyalty by making customers feel valued and understood.
Why Personalization Matters
Customers today expect more than generic responses. They seek interactions that address their unique needs and preferences. Studies reveal that personalized support significantly impacts customer satisfaction. For instance:
Evidence | Description |
---|---|
44% of consumers | Changed their primary financial service provider due to poor personalization (BCG, 2022). |
20% higher satisfaction | Customers receiving personalized service demonstrate significantly higher satisfaction rates. |
7 times more likely | Customers are more likely to respond positively to offers from their bank when they receive personalized service. |
These findings underscore the importance of integrating personalization into customer interactions. Businesses that fail to meet these expectations risk losing customers to competitors who prioritize tailored experiences.
How RAG Enhances Personalization
RAG systems enable businesses to deliver personalized support by dynamically retrieving and analyzing customer-specific data. This capability allows for:
- Tailored Recommendations: RAG systems suggest products or services based on individual preferences and purchase history. For example, an e-commerce platform can recommend items that align with a customer's browsing behavior.
- Context-Aware Responses: By considering past interactions, RAG systems ensure that responses remain relevant and contextually appropriate. This reduces redundancy and enhances the overall experience.
- Sentiment-Driven Interactions: Advanced sentiment analysis enables RAG systems to adjust their tone and approach based on the customer's emotional state. A frustrated customer might receive empathetic responses, while a satisfied one could be offered upselling opportunities.
Real-World Impact of Personalization
The benefits of personalization extend beyond customer satisfaction. Companies that implement advanced personalization strategies often see measurable improvements in key performance metrics. For example:
Evidence | Description |
---|---|
23% increase | Banks implementing advanced personalization strategies see an average increase in customer satisfaction scores. |
20 points | Net Promoter Scores (NPS) jump by up to 20 points with advanced personalization (KPMG, 2022). |
Additionally, Forbes reports that82% of guests who received personalized service reported higher satisfaction levels. These statistics highlight the transformative potential of personalization in customer support.
Building Long-Term Loyalty Through Personalization
Personalized interactions do more than improve immediate satisfaction; they build long-term loyalty. Customers who feel understood and valued are more likely to return and recommend the business to others. RAG-based customer service plays a pivotal role in achieving this by consistently delivering responses that align with individual needs.
For example, a subscription-based service using RAG technology can analyze usage patterns to offer tailored renewal plans or suggest upgrades. This proactive approach not only enhances the customer experience but also strengthens the relationship between the customer and the brand.
Did You Know?: Customers are 7 times more likely to engage with personalized offers, making personalization a critical strategy for driving engagement and retention.By integrating RAG systems into their customer service platforms, businesses can meet the growing demand for personalization, ensuring higher satisfaction rates and fostering lasting loyalty.
Real-World Applications of RAG in Customer Support
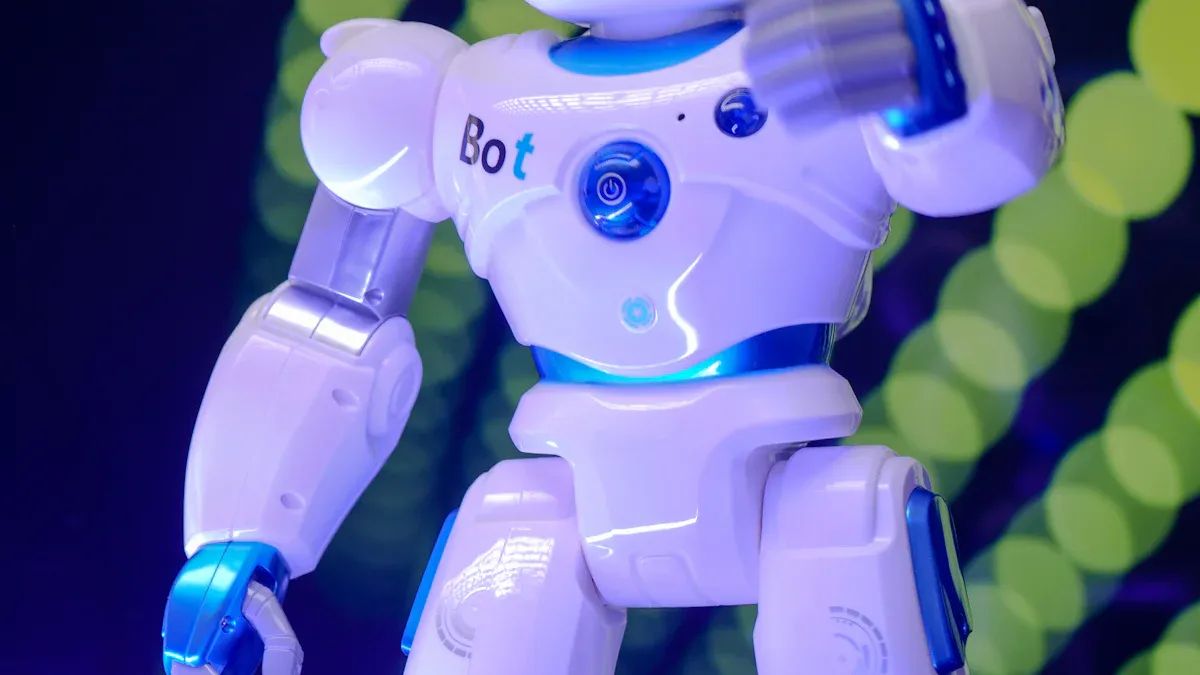
RAG-Powered Chatbots for E-Commerce
E-commerce platforms increasingly rely on RAG-powered AI to enhance customer support chatbots. These chatbots provide real-time responses by retrieving accurate product information, order details, and shipping updates. Unlike traditional systems, RAG-powered chatbots dynamically fetch data from external sources, ensuring customers receive up-to-date answers. For instance, a customer querying a product's availability can instantly receive accurate stock information, improving their shopping experience.
RAG-powered chatbots also excel in handling complex queries. By integrating multi-step retrieval pipelines, they achieve over 50% higher accuracy in resolving customer issues, as demonstrated by the FRAMES evaluation. This capability reduces the need for human intervention, enabling businesses to manage high query volumes efficiently. Additionally,RAGTruth's focus on hallucination detection ensures that responses remain reliable, further enhancing customer trust.
Pro Tip: E-commerce businesses can boost customer satisfaction by deploying RAG-powered chatbots to provide personalized product recommendations based on browsing history.Enhancing Technical Support with RAG in SaaS
Software-as-a-Service (SaaS) companies face unique challenges in technical support. RAG-based systems address these challenges by offering real-time solutions to technical issues. These systems retrieve relevant documentation, FAQs, and troubleshooting guides, enabling faster resolution of customer problems. For example, a SaaS provider using RAG can reduce issue resolution times by 28.6%, as shown in operational efficiency metrics.
RAG's adaptability allows SaaS companies to update their support systems without extensive retraining. This ensures that customers always receive accurate information, even when software updates occur. By integrating RAG, SaaS providers can enhance their support capabilities while reducing operational costs.
Integrating RAG with Virtual Assistants for Better Service
Virtual assistants powered by RAG deliver superior customer service by combining real-time data retrieval with advanced AI capabilities. These assistants provide accurate and context-aware responses, ensuring a seamless user experience. For instance, a virtual assistant integrated with RAG can dynamically fetch new information, eliminating the need for manual updates.
Metrics | Traditional AI Model | RAG – Enhanced AI |
---|---|---|
Response Accuracy | Prone to outdated or hallucinated facts | More accurate and up-to-date |
Operational Efficiency | Slower issue resolution times | Median time to resolve issuesreduced by 28.6% |
Adaptability | Requires re-training for new data | Can dynamically fetch new information |
These improvements make RAG-powered virtual assistants indispensable for businesses aiming toenhance customer interactions. By leveraging RAG, companies can provide personalized and efficient support, fostering long-term customer loyalty.
RAG transforms customer service by enhancing efficiency and accuracy. Its ability to retrieve real-time data and generate context-aware responses ensures faster resolution times and reduced errors. Businesses adopting RAG systems benefit from improved decision-making, streamlined processes, andlower operational costs.
Benefit | Description |
---|---|
Enhanced Decision-Making | RAG systems improve decision-making by integrating human and AI inputs for more accurate responses. |
Improved Response Accuracy | The systems optimize query formulation, leading to more context-aware answers. |
Reduced Average Handling Time | By streamlining processes, RAG systems decrease the time agents spend on each customer interaction. |
Lower Operational Costs | The efficiency gained from RAG systems contributes to significant cost savings in customer service. |
Businesses aiming to stay competitive should explore RAG-based solutions. These systems not only address immediate customer needs but also lay the foundation for scalable, personalized support that fosters long-term loyalty.
Note: Companies investing in RAG technology position themselves as leaders in customer service innovation, ensuring sustainable growth and satisfaction.FAQ
What is RAG technology in customer service?
RAG, or Retrieval-Augmented Generation, combines real-time data retrieval with AI-driven text generation. It enhances customer service by providing accurate, context-aware responses, improving efficiency and personalization.
How does RAG improve response times?
RAG systems retrieve real-time data, enabling instant responses. This reduces wait times and enhances customer satisfaction by delivering precise answers quickly, unlike traditional methods that rely on manual processes.
Can RAG handle complex customer queries?
Yes, RAG excels in managing complex queries. It uses advanced AI to analyze and retrieve relevant information, ensuring accurate and contextually appropriate responses, even for intricate customer issues.
Is RAG suitable for small businesses?
Absolutely! RAG systems offer scalable solutions, reducing operational costs and improving efficiency. Small businesses can benefit from 24/7 support without the need for extensive human resources.
How does RAG ensure personalized customer interactions?
RAG analyzes customer data, such as past interactions and preferences, to generate personalized responses. This approach enhances customer satisfaction by delivering tailored experiences that resonate with individual needs.
Previous Blogs
How RAG Improves Customer Service Efficiency and Accuracy
AG-based customer service boosts efficiency and accuracy by combining real-time data retrieval with AI, ensuring precise, context-aware responses for customers.
A Comprehensive Guide to Enterprise RAG Implementation Success
Enterprise RAG implementation guide: Avoid pitfalls in self-development, analyze top frameworks, and configure systems for scalability and success.