DeepSeek Local AI Deployment + RAG: Boost Performance & Security
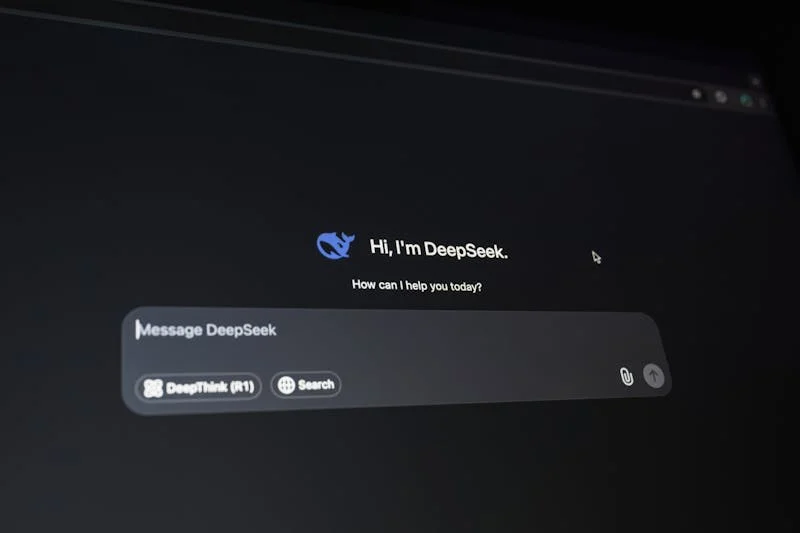
Introduction: Why Local Deployment and RAG Are Key to Optimizing AI Performance
In today's fast-paced digital landscape, the demand for scalable and efficient AI systems is growing exponentially. Businesses across industries are leveraging AI to drive innovation, improve decision-making, and deliver personalized experiences. However, as AI applications become more complex, the need for optimized performance, data privacy, and real-time processing has never been more critical.
This is where local deployment and Retrieval-Augmented Generation (RAG) come into play. Local deployment allows organizations to run AI models directly on their own infrastructure, ensuring greater control, security, and speed. RAG, on the other hand, is a revolutionary approach that combines data retrieval with generative AI, significantly enhancing the relevance and accuracy of AI-generated outputs.
When these two technologies are combined—specifically, deploying Deepseek locally with RAG—the result is a powerful synergy that can transform how AI systems perform. This blog will explore why this combination is a game-changer for AI performance, particularly for businesses in the US and China, where AI adoption is rapidly accelerating.
What Makes Deepseek Stand Out in Local AI Deployment
Deepseek is a cutting-edge AI platform designed for efficient data retrieval and processing. Its unique architecture makes it particularly well-suited for local deployment, offering several key advantages:
- Privacy and Security: By deploying Deepseek locally, organizations can ensure that sensitive data never leaves their infrastructure. This is especially important in industries like healthcare, finance, and government, where data privacy is paramount.
- Control and Customization: Local deployment gives businesses full control over their AI systems, allowing them to tailor models to their specific needs and integrate them seamlessly with existing workflows.
- Speed and Efficiency: Running Deepseek on local hardware eliminates latency issues associated with cloud-based solutions, enabling real-time data processing and faster decision-making.
These benefits make Deepseek an ideal choice for businesses looking to maximize the performance of their AI systems while maintaining data integrity and compliance.
RAG: A Revolutionary Approach to AI that Enhances Data Retrieval and Generation
Retrieval-Augmented Generation (RAG) is a groundbreaking technique that bridges the gap between data retrieval and generative AI. Traditional generative models, while powerful, often struggle with producing accurate and contextually relevant outputs. RAG addresses this limitation by integrating a retrieval mechanism that fetches relevant data from external sources before generating a response.
Here's how RAG works:
- Data Retrieval: When a query is received, the system retrieves relevant information from a predefined knowledge base or dataset.
- Contextual Generation: The retrieved data is then fed into a generative model, which uses it to produce a highly accurate and contextually relevant response.
This approach not only improves the quality of AI-generated content but also ensures that the outputs are grounded in factual information. For example, in customer support applications, RAG can help AI systems provide more accurate and helpful responses by referencing up-to-date product manuals or FAQs.
The Synergy Between Deepseek and RAG: A Powerful Combination for AI Performance
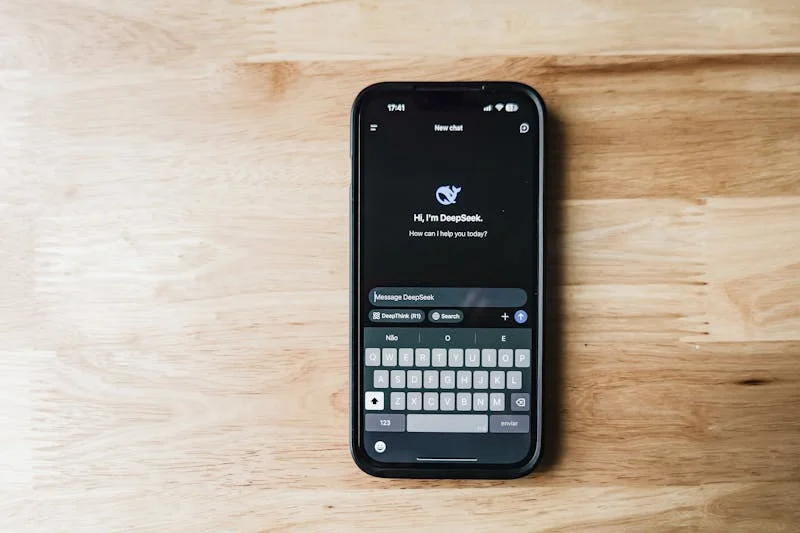
When Deepseek is deployed locally and integrated with RAG, the result is a robust AI system that excels in both data retrieval and generation. Here's how this synergy works:
- Enhanced Data Retrieval: Deepseek's efficient data retrieval capabilities ensure that the most relevant information is fetched quickly, even from large and complex datasets.
- High-Quality Generation: RAG leverages the retrieved data to generate outputs that are not only accurate but also contextually rich and relevant.
This combination is particularly effective in use cases such as:
- Personalized Recommendations: E-commerce platforms can use Deepseek and RAG to analyze user behavior and generate personalized product recommendations in real time.
- Knowledge Extraction: Enterprises can deploy this system to extract actionable insights from vast amounts of unstructured data, such as research papers or legal documents.
- Customer Support: AI-powered chatbots can provide faster and more accurate responses by retrieving information from a centralized knowledge base.
Technical Insights: Deploying Deepseek Locally and Integrating RAG
Deploying Deepseek locally and integrating it with RAG requires careful planning and execution. Here's a step-by-step guide to help you get started:
- System Requirements: Ensure that your local infrastructure meets the hardware and software requirements for running Deepseek and RAG. This typically includes high-performance GPUs, sufficient storage, and compatible operating systems.
- Installation and Setup: Download and install Deepseek on your local server. Follow the official documentation to configure the system and set up the necessary dependencies.
- Integrating RAG: Once Deepseek is up and running, integrate RAG by configuring the retrieval and generation modules. This involves setting up the knowledge base, fine-tuning the generative model, and ensuring seamless communication between the two components.
- Testing and Optimization: Test the system thoroughly to ensure that it performs as expected. Optimize the configuration based on your specific use case and performance requirements.
Challenges in Local Deployment and RAG Integration
While the benefits of local deployment and RAG integration are significant, there are also challenges to consider:
- Hardware Limitations: Running AI models locally requires substantial computational resources, which can be costly and difficult to scale.
- Resource Allocation: Efficiently allocating resources to ensure smooth performance can be complex, especially in environments with fluctuating workloads.
- Data Synchronization: Keeping the knowledge base up to date and ensuring data consistency across the system can be challenging.
- Troubleshooting: Identifying and resolving technical issues during deployment requires expertise and careful monitoring.
Despite these challenges, the long-term benefits of local deployment and RAG integration far outweigh the initial hurdles, making it a worthwhile investment for businesses aiming to optimize their AI systems.
The Future of Local AI Deployment with Deepseek and RAG: Opportunities and Innovations
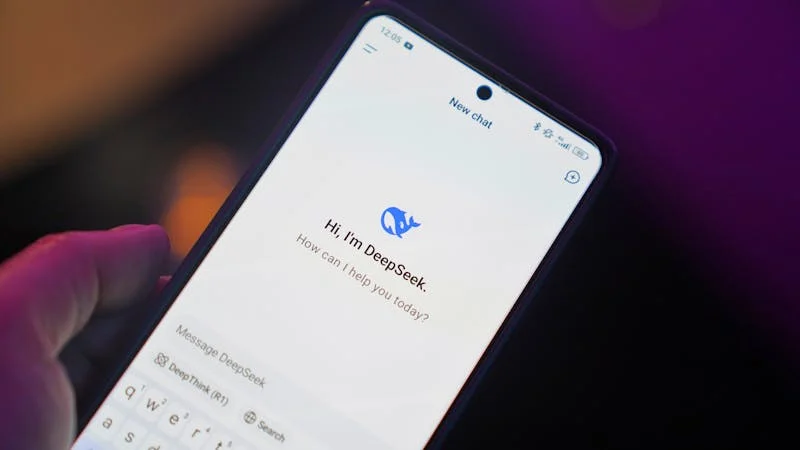
The future of local AI deployment with Deepseek and RAG is bright, with several emerging technologies poised to enhance its capabilities further:
- Edge Computing: By bringing AI processing closer to the data source, edge computing can reduce latency and improve real-time performance, making it an ideal complement to local deployment.
- Federated Learning: This approach allows AI models to be trained across multiple decentralized devices while keeping data localized, enhancing privacy and scalability.
- Advancements in AI Models: As generative models continue to evolve, their ability to produce high-quality outputs will improve, further enhancing the effectiveness of RAG.
These innovations will enable businesses to unlock new possibilities in AI applications, from autonomous systems to advanced analytics, while maintaining the benefits of local deployment.
Conclusion: Unlocking the Full Potential of Your AI with Deepseek and RAG
In a world where AI is becoming increasingly integral to business success, optimizing its performance is no longer optional—it's essential. By deploying Deepseek locally and integrating it with RAG, organizations can achieve unparalleled levels of accuracy, speed, and control in their AI systems.
This powerful combination not only addresses the challenges of data privacy and real-time processing but also opens up new opportunities for innovation and growth. Whether you're a business in the US or China, adopting this strategy can help you stay ahead of the curve and future-proof your AI investments.
The time to act is now. Embrace the potential of Deepseek and RAG, and unlock the full capabilities of your AI systems to drive long-term success.
Previous Blogs
DeepSeek R1 on AMD Radeon GPUs and Other Hardware: A Performance Breakdown
Discover how AMD Radeon GPUs and other hardware perform in DeepSeek R1 benchmarks. Learn about the latest tools and strategies for leveraging AI to boost sales, engage customers, and drive revenue.
DeepSeek R1 vs ChatGPT o1: Which AI Model Stands Out?
Compare DeepSeek-R1 vs. ChatGPT-o1 to find out which AI model excels in reasoning, conversational tasks, cost-effectiveness, and user engagement.