Why Human-AI Collaboration Faces Cognitive and Technical Barriers
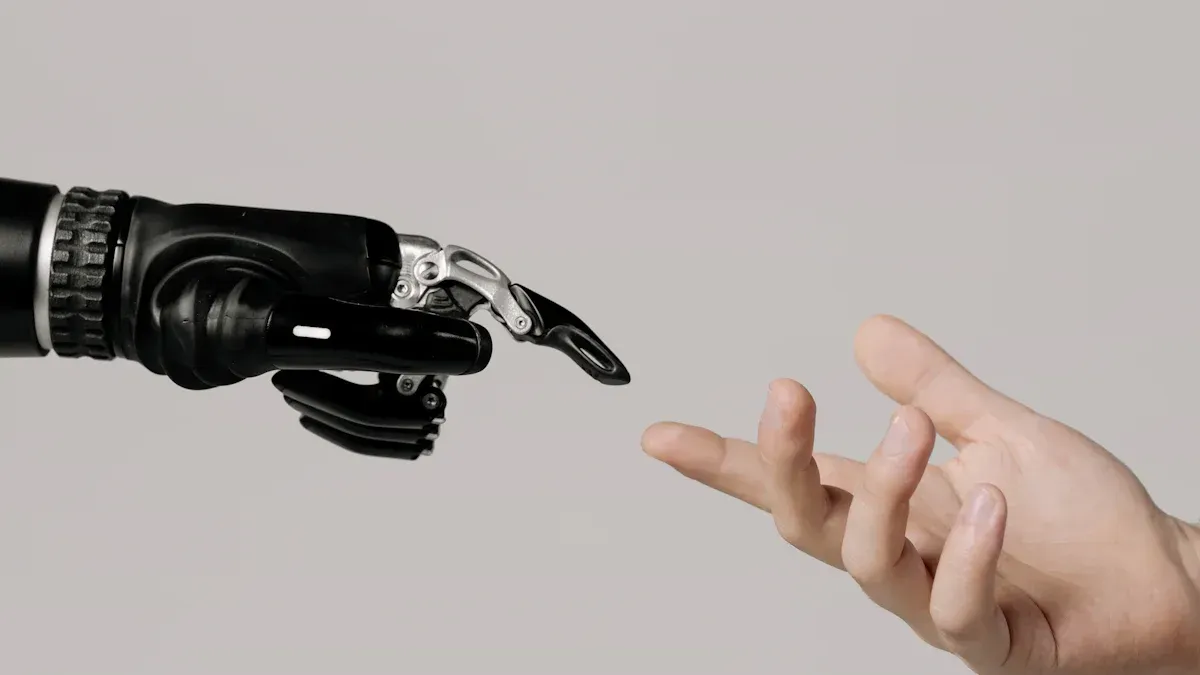
Human-AI collaboration plays a vital role in shaping modern technology. You rely on AI systems to enhance productivity and solve complex problems. However, challenges in human-AI agent collaboration often disrupt this partnership. These challenges arise from differences in how humans and AI think, work, and interact. Addressing them can unlock new possibilities.
Key Takeaways
- Learn how humans and AI think differently. Mixing human instincts with AI's logic can improve choices.
- Trust AI more by making it clear how it works. Simple reasons for AI's actions make people trust and work with it better.
- Know what AI can't do to avoid problems. Understanding limits stops too much trust in AI and helps people stay in control.
Cognitive Challenges in Human-AI Agent Collaboration
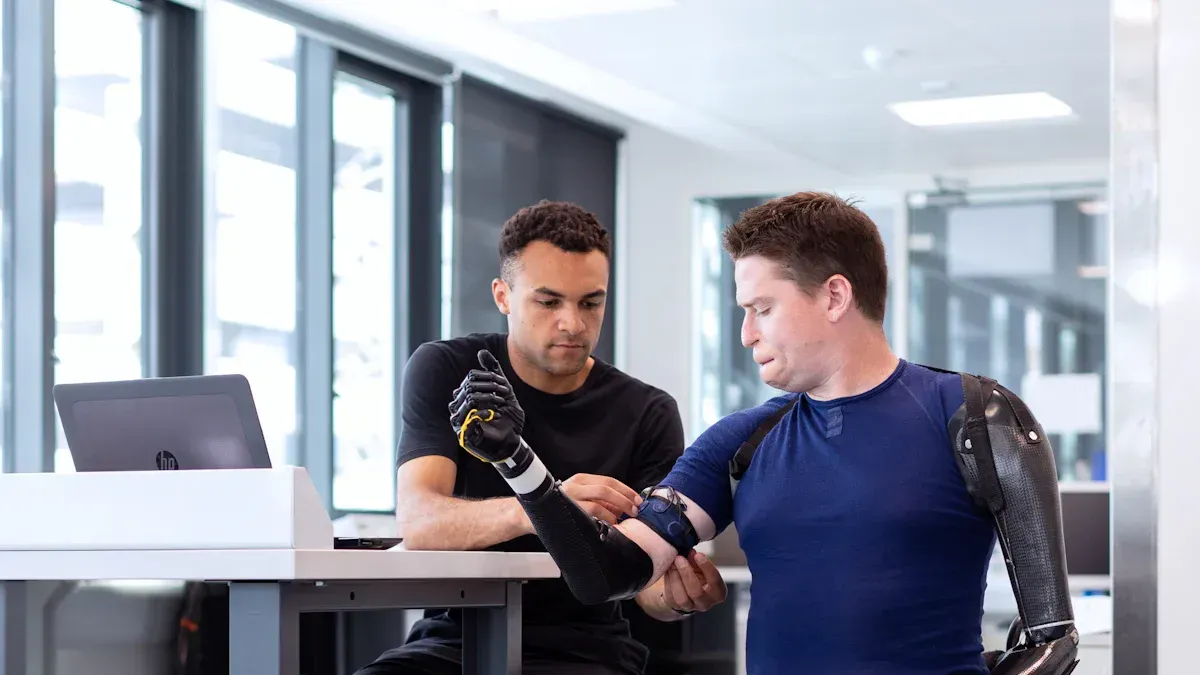
Differences in Reasoning and Decision-Making
Humans and AI approach reasoning and decision-making differently. You rely on intuition, creativity, and experience to make decisions, while AI depends on data-driven algorithms and predefined rules. For example, studies show that combining human intuition with AI's analytical power can improve outcomes in fields like healthcare and scientific research. However, communication barriers often arise because AI lacks the ability to explain its reasoning in a way you can easily understand. This disconnect creates challenges in human-AI agent collaboration, particularly when decisions require a blend of logic and emotional intelligence.
Trust Issues and Transparency in AI Systems
Trust plays a critical role in any collaboration, including those involving AI. Transparency gaps in AI systems often undermine trust. For instance, surveys reveal that insufficient explanations of AI decisions hinder accountability and transparency. Users may feel uneasy when they cannot understand how an AI system reaches its conclusions. Additionally, inadequate data documentation and limited operational clarity further erode trust. These issues highlight the importance of designing AI systems that prioritize clear communication and user confidence.
Human Adaptability and Resistance to Change
Adapting to AI systems can be challenging for many people. You may find it difficult to adjust to new workflows or technologies, especially when they conflict with your established habits. Metrics like adaptability scores and task completion times illustrate how humans respond to AI integration. For example, when AI systems focus on efficiency, they may overlook human goals like effective teaching or task satisfaction. This misalignment can lead to resistance, slowing down the adoption of AI in various environments.
Technical Challenges in Human-AI Agent Collaboration
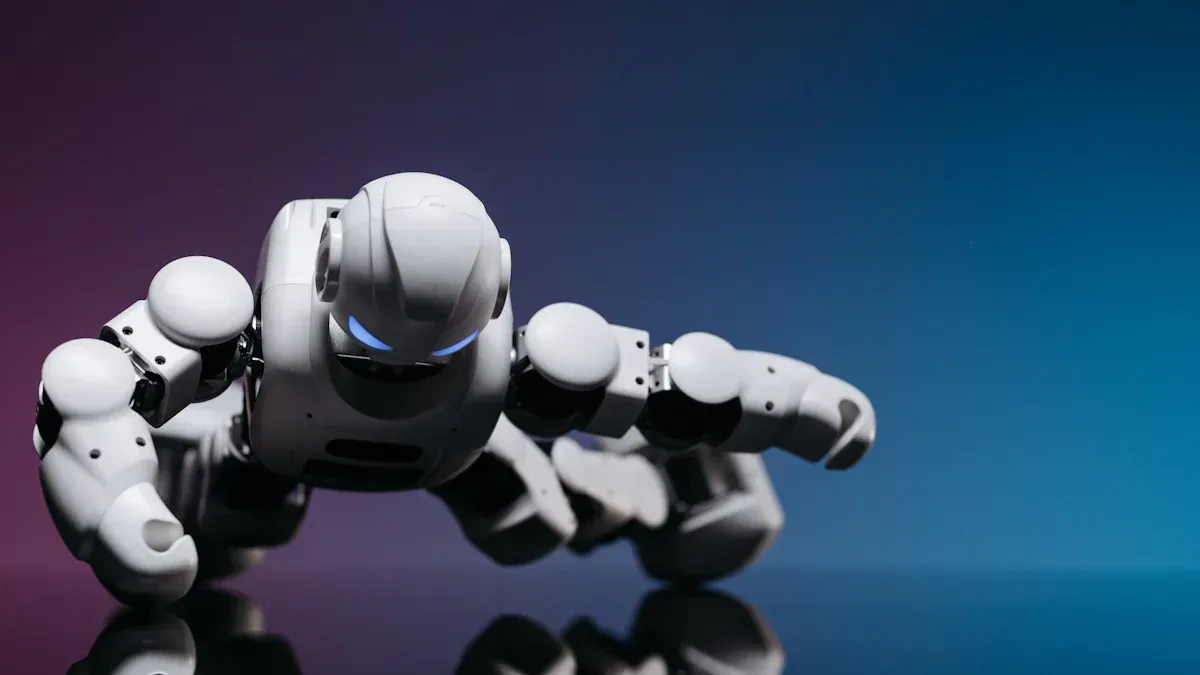
Limitations in AI Design and Functionality
AI systems often struggle with tasks that require flexibility or nuanced understanding. For example, benchmarks like GAIA reveal that advanced AI systems excel at complex tasks but fail at simpler ones. This inconsistency highlights a significant limitation in AI design. Similarly, evaluations like Humanity’s Last Exam show that AI cannot yet match human expertise in answering challenging questions. These performance gaps stem from the rigid nature of AI algorithms, which lack the creativity and adaptability you bring to problem-solving. Addressing these limitations requires ongoing improvements in AI architecture and functionality.
Data Quality, Bias, and Ethical Concerns
The quality of data used to train AI systems directly impacts their performance. Poor data quality can lead to catastrophic outcomes. For instance, a 2017 self-driving car accident in Florida occurred because the AI failed to detect a white truck against a bright sky due to inaccurate image annotations. Bias in datasets also poses ethical challenges. Amazon’s AI recruiting tool, for example, exhibited gender bias because it relied on historical hiring data that favored men. These cases emphasize the importance of using unbiased, high-quality data to prevent harmful consequences and ensure ethical AI deployment.
Integration Issues Between AI Systems and Human Workflows
Integrating AI into existing workflows presents significant challenges. Organizations often face hurdles like data compatibility and system architecture modifications. Studies show that these issues can disrupt workflow effectiveness and create resistance among professionals. For example, mental health AI systems have raised ethical concerns and varying levels of trust, complicating their adoption. To overcome these challenges, you can adopt strategies like regular AI audits and human-in-the-loop approaches. These methods ensure that AI systems align with human needs and enhance collaboration.
The Interplay of Cognitive and Technical Challenges
How Cognitive Barriers Exacerbate Technical Limitations
Cognitive barriers often amplify the technical limitations of AI systems. For example, human biases can lead to over-reliance on AI, especially in situations where human judgment is critical. You might trust AI systems to make decisions without questioning their accuracy, which can result in errors when the AI encounters unfamiliar scenarios. AI systems also depend heavily on high-quality, representative data. When this data is flawed, the system's performance suffers, and human oversight becomes essential to mitigate these issues.
The collaboration between humans and AI highlights the need for ethical reasoning and contextual understanding. These are areas where AI lacks intuition, but your cognitive abilities can fill the gap. This interplay demonstrates how addressing cognitive barriers, such as over-reliance or misunderstanding AI capabilities, can help overcome technical challenges.
How Technical Barriers Undermine Human Trust and Collaboration
Technical barriers, like poor AI design or lack of transparency, can erode your trust in AI systems. When an AI system fails to explain its decisions clearly, you may hesitate to rely on it. For instance, algorithms that lack transparency often create confusion, making it harder for you to collaborate effectively. Ethical concerns, such as biased data, further complicate this relationship.
To rebuild trust, AI systems must prioritize user satisfaction and operational clarity. A study analyzing collaboration metrics—accuracy, efficiency, and satisfaction—shows that clear communication protocols improve human-AI partnerships. This reinforces the importance of designing AI systems that align with your expectations and needs.
The Feedback Loop Between Cognitive and Technical Challenges
Cognitive and technical challenges in human-AI collaboration often create a feedback loop. For instance, when AI systems fail due to technical flaws, your confidence in their capabilities decreases. This lack of trust can lead to resistance, which further limits the effectiveness of AI integration. On the other hand, cognitive barriers, such as misunderstanding AI's limitations, can exacerbate technical issues.
The inherent limitations of big data highlight this feedback loop. Human judgment is crucial to ensure that algorithms function effectively. By combining your cognitive strengths with AI's computational power, you can address these intertwined challenges. This symbiosis enhances decision-making and fosters a more productive collaboration.
Tip: To improve collaboration, focus on understanding AI's capabilities and limitations while advocating for transparent and user-friendly systems.Human-AI collaboration faces intertwined challenges in human-AI agent collaboration that demand attention. Addressing these issues requires improving AI design, fostering trust, and enhancing adaptability. For example:
- Automation bias can hinder collaboration.
- Integration issues disrupt workflows.
- Training programs and transparent systems build trust.
Overcoming these barriers unlocks the full potential of human-AI partnerships.
FAQ
What is the biggest challenge in human-AI collaboration?
The biggest challenge is the lack of trust. You may find it hard to rely on AI systems when they fail to explain their decisions clearly.
How can you improve trust in AI systems?
You can improve trust by advocating for transparent AI designs. Systems that explain their processes and decisions foster confidence and better collaboration.
Why does AI struggle with ethical concerns?
AI struggles with ethics because it relies on biased or incomplete data. You must ensure datasets are diverse and representative to avoid harmful outcomes.
Note: Understanding AI's limitations helps you collaborate effectively and avoid common pitfalls.Previous Blogs
How RAG Improves Customer Service Efficiency and Accuracy
AG-based customer service boosts efficiency and accuracy by combining real-time data retrieval with AI, ensuring precise, context-aware responses for customers.
A Comprehensive Guide to Enterprise RAG Implementation Success
Enterprise RAG implementation guide: Avoid pitfalls in self-development, analyze top frameworks, and configure systems for scalability and success.