Reinforcement Learning in India: Opportunities, Challenges, and Applications
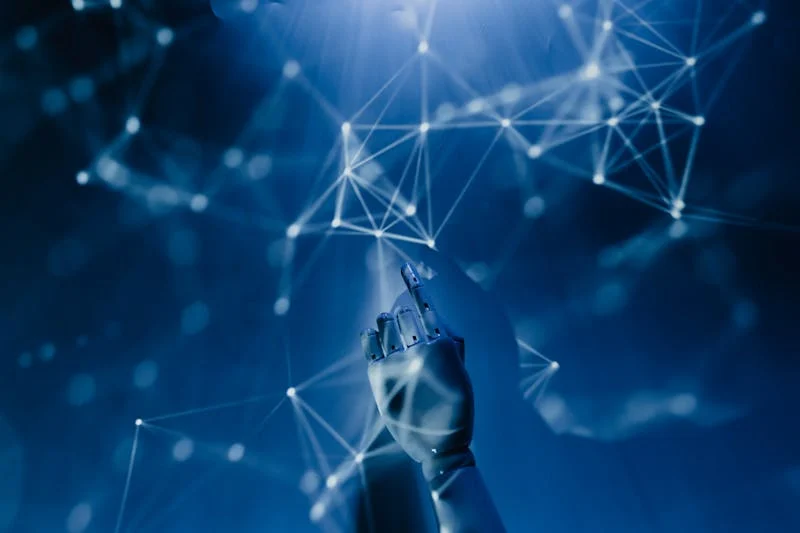
Introduction
Reinforcement Learning (RL) is a cutting-edge branch of artificial intelligence (AI) that focuses on training agents to make sequential decisions by interacting with an environment. Unlike supervised and unsupervised learning, RL relies on trial and error, where agents learn from rewards and penalties to optimize their behavior. This approach has gained immense traction globally, powering advancements in autonomous systems, robotics, and personalized recommendations.
India, with its rapidly growing tech ecosystem, is emerging as a key market for RL adoption. With a thriving start-up culture, government-backed AI initiatives, and a vast pool of skilled professionals, India is uniquely positioned to leverage RL for solving complex challenges in sectors like healthcare, agriculture, and logistics. This blog explores the opportunities, challenges, and applications of reinforcement learning in India, highlighting its potential to transform industries and drive innovation.
1. Understanding Reinforcement Learning
1.1 What is Reinforcement Learning?
Reinforcement Learning (RL) is a machine learning paradigm where an agent learns to make decisions by interacting with an environment. The agent takes actions, receives feedback in the form of rewards or penalties, and adjusts its strategy to maximize cumulative rewards over time.
Key Differences Between RL and Other Machine Learning Paradigms
- Supervised Learning: Requires labeled data to train models, while RL learns from interactions.
- Unsupervised Learning: Focuses on finding patterns in unlabeled data, whereas RL emphasizes decision-making.
- RL's Unique Strength: Ability to handle sequential decision-making problems, such as game playing or resource allocation.
1.2 How RL Works
Agents, Environments, States, Actions, and Rewards
- Agent: The decision-maker that interacts with the environment.
- Environment: The external system the agent operates in.
- State: The current situation of the environment.
- Action: A decision taken by the agent.
- Reward: Feedback received after an action, guiding the agent's learning.
Exploration vs. Exploitation
- Exploration: Trying new actions to discover their outcomes.
- Exploitation: Leveraging known actions to maximize rewards.Balancing these two is crucial for effective RL.
1.3 Popular RL Algorithms
Q-Learning
A model-free algorithm that learns the value of actions in specific states.
Deep Q-Networks (DQN)
Combines Q-learning with deep neural networks to handle complex environments.
Policy Gradient Methods
Directly optimize the policy (strategy) of the agent using gradient ascent.
Real-World Examples
- AlphaGo: Used RL to master the game of Go.
- Robotics: RL powers autonomous robots in manufacturing and logistics.
2. The Growing Importance of RL in India
2.1 India's AI and Machine Learning Landscape
India is rapidly adopting AI technologies, driven by its tech-savvy population and government initiatives like the National AI Strategy. The country is home to over 1,500 AI start-ups, making it a hub for innovation.
2.2 Why RL is Gaining Traction in India
Relevance to Key Industries
- Healthcare: RL can optimize treatment plans and resource allocation.
- Agriculture: Enables precision farming and smarter irrigation systems.
- Logistics: Improves supply chain efficiency and traffic management.
Role of Start-Ups and Tech Hubs
Indian start-ups are leveraging RL to develop innovative solutions, supported by tech hubs like Bengaluru, Hyderabad, and Pune.
2.3 Opportunities for RL Adoption in Emerging Sectors
Supply Chain Optimization
RL can streamline logistics, reduce costs, and improve delivery times.
Education Systems
Personalized learning platforms powered by RL can enhance student outcomes.
Customer Experience
RL-driven recommendation systems can boost engagement in e-commerce and entertainment.
3. Applications of RL in Key Indian Industries
3.1 Healthcare Sector
Personalized Treatment Plans
RL models can tailor treatments based on patient data, improving outcomes.
Enhancing Diagnostic Tools
Predictive analytics powered by RL can aid in early disease detection.
3.2 Agriculture and Rural Development
Precision Farming
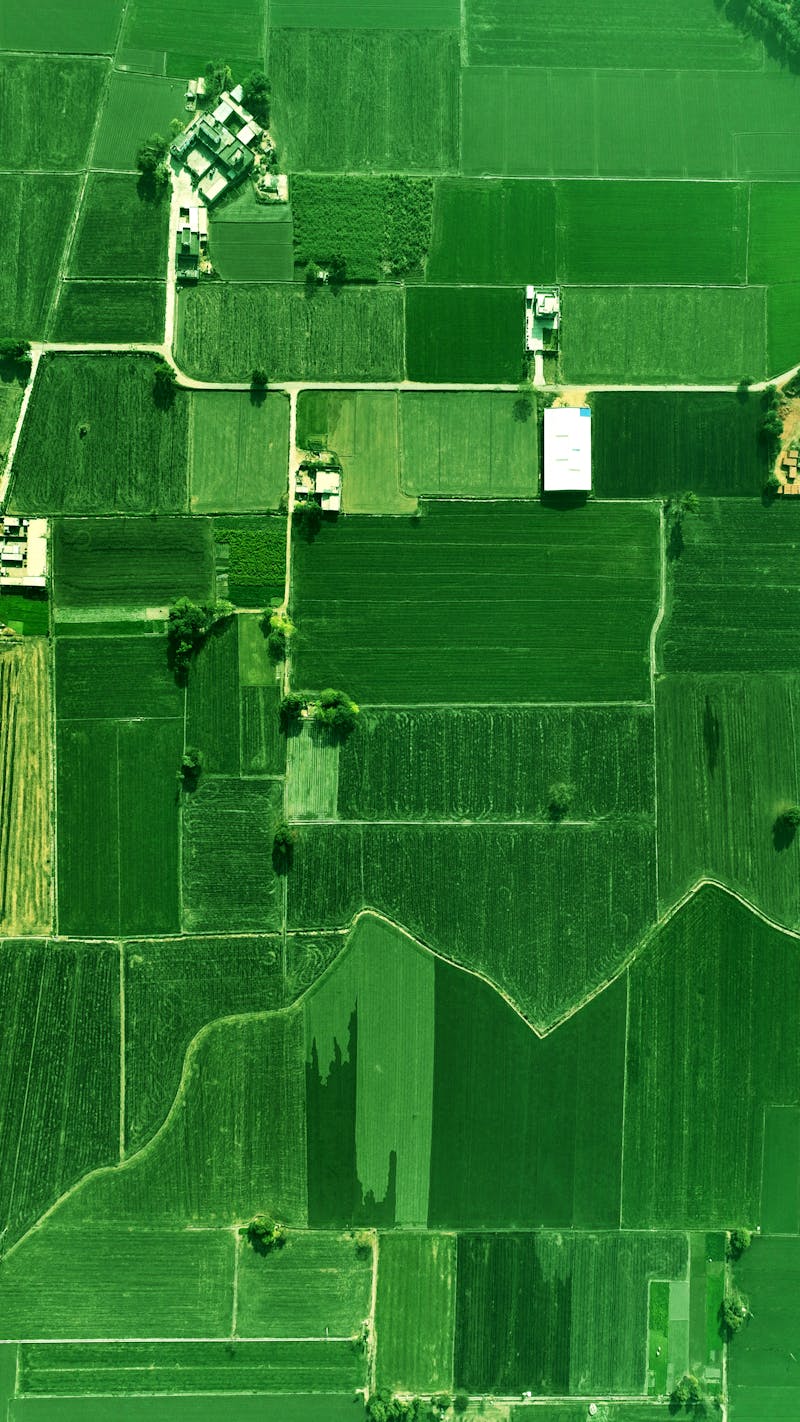
RL algorithms optimize crop management, reducing waste and increasing yields.
Smarter Irrigation Systems
RL helps manage water resources efficiently, addressing water scarcity.
3.3 Transportation and Logistics
Optimizing Traffic Flow
RL-powered systems can reduce congestion in Indian cities.
Autonomous Vehicles
RL is driving research in self-driving cars, with potential applications in India.
3.4 Financial Services
Fraud Detection
RL models can identify fraudulent transactions in real-time.
Personalized Financial Products
RL enhances customer experience by offering tailored financial solutions.
4. Challenges in Adopting Reinforcement Learning in India
4.1 Limited Infrastructure for Advanced AI Research
High-Performance Computing
India needs more investment in computing resources to support RL research.
Access to Quality Datasets
The lack of standardized datasets hinders the development of robust RL models.
4.2 Talent Shortage in AI and ML Fields
Demand-Supply Gap
There is a shortage of skilled professionals in RL and related fields.
Upskilling Initiatives
Government and private sector efforts are needed to train the workforce in RL.
4.3 Ethical Concerns and Regulatory Challenges
Bias in RL Models
Ensuring fairness and transparency in RL algorithms is critical.
AI Governance
India needs robust frameworks to regulate AI and RL applications.
5. Case Studies: Successful RL Implementations in India
5.1 Start-Up Innovations in RL
Example: CropIn
An Indian agri-tech start-up using RL for precision farming solutions.
5.2 Academic Contributions to RL Research
Key Research Papers
Indian universities are publishing cutting-edge research in RL.
5.3 Government-Led Projects Leveraging RL
Smart City Initiatives
RL is being used to optimize traffic and energy management in smart cities.
6. The Future of Reinforcement Learning in India
6.1 Emerging Trends in RL Development
Integration with IoT and Blockchain
Combining RL with IoT and blockchain can unlock new possibilities.
6.2 Building a Robust Ecosystem for RL Growth
Collaboration Between Stakeholders
Academia, industry, and government must work together to foster RL innovation.
6.3 Long-Term Vision: India as a Global Leader in RL Innovation
Steps Needed
Investments in research, infrastructure, and talent development are essential.
Conclusion
Reinforcement Learning holds immense potential to transform industries and drive innovation in India. From healthcare and agriculture to logistics and finance, RL can address some of the country's most pressing challenges. However, realizing this potential requires overcoming infrastructure gaps, addressing talent shortages, and establishing robust governance frameworks. By investing in RL research and applications, India can position itself as a global leader in this transformative technology.
The time is ripe for stakeholders—government, industry, and academia—to collaborate and harness the power of reinforcement learning for a brighter, smarter future.
FAQ
1. What is Reinforcement Learning (RL)?
Reinforcement Learning is a machine learning paradigm where an agent learns to make decisions by interacting with an environment, receiving rewards or penalties, and optimizing its behavior to maximize cumulative rewards.
2. How is RL different from other machine learning methods?
Unlike supervised learning (which uses labeled data) and unsupervised learning (which finds patterns in unlabeled data), RL focuses on sequential decision-making through trial and error.
3. Why is RL important for India?
India's diverse industries, such as healthcare, agriculture, and logistics, can benefit from RL's ability to optimize complex processes and solve real-world problems.
4. What are the challenges of adopting RL in India?
Key challenges include limited infrastructure for advanced research, a shortage of skilled professionals, and ethical concerns related to bias and governance.
5. What are some real-world applications of RL in India?
RL is being used in precision farming, traffic optimization, personalized healthcare, and fraud detection, among other applications.
6. How can India become a global leader in RL?
By investing in research, infrastructure, and talent development, and fostering collaboration between academia, industry, and government, India can position itself as a leader in RL innovation.
7. What role do start-ups play in RL adoption in India?
Indian start-ups are driving innovation by developing RL-based solutions for unique challenges in sectors like agriculture, healthcare, and logistics.
8. Are there any government initiatives supporting RL in India?
Yes, initiatives like the National AI Strategy and smart city projects are promoting the adoption of RL and other AI technologies in India.
9. What is the future of RL in India?
The future looks promising, with emerging trends like the integration of RL with IoT and blockchain, and a growing focus on building a robust ecosystem for RL growth.
10. How can individuals contribute to RL advancements in India?
By pursuing education and research in AI and RL, participating in upskilling programs, and collaborating with industry and academia, individuals can contribute to India's RL ecosystem.
Previous Blogs
How RAG Improves Customer Service Efficiency and Accuracy
AG-based customer service boosts efficiency and accuracy by combining real-time data retrieval with AI, ensuring precise, context-aware responses for customers.
A Comprehensive Guide to Enterprise RAG Implementation Success
Enterprise RAG implementation guide: Avoid pitfalls in self-development, analyze top frameworks, and configure systems for scalability and success.