How Multimodal AI Agents Are Revolutionizing Healthcare Diagnostics
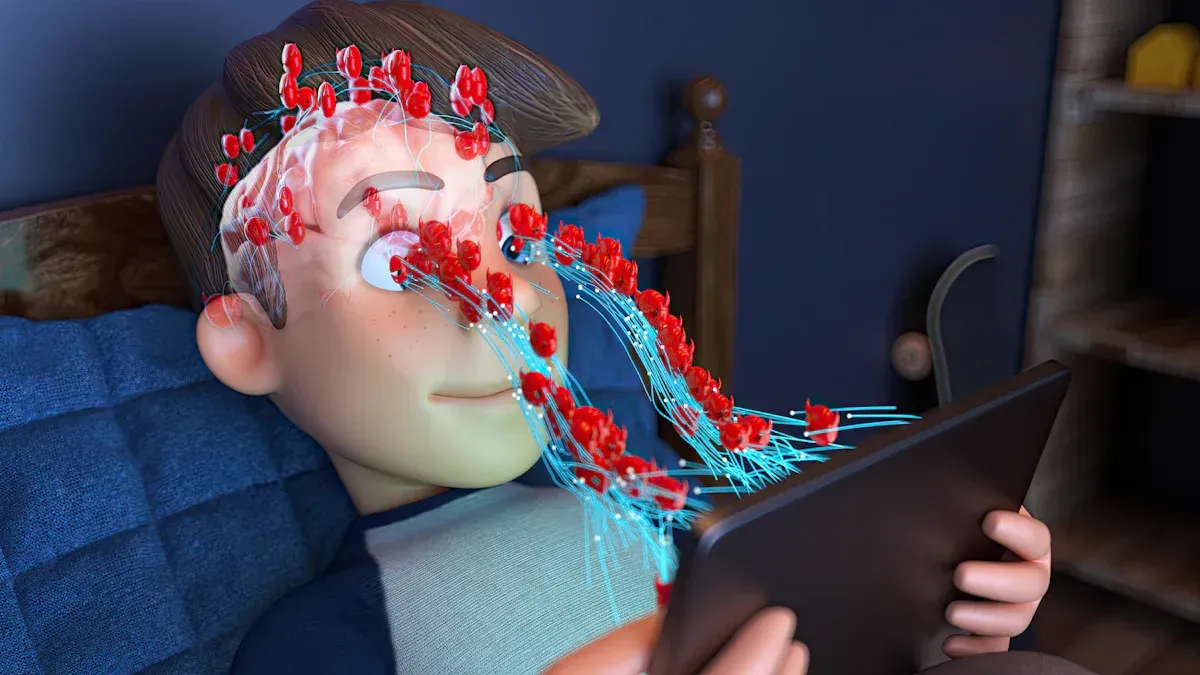
Healthcare diagnostics are undergoing a transformation thanks to multimodal AI agents in healthcare diagnostics. These advanced systems combine data from multiple sources, such as medical images, electronic health records, and lab results, to deliver precise and timely diagnoses. For example, during the COVID-19 pandemic, multimodal AI agents in healthcare diagnostics achieved over 90% diagnostic accuracy by integrating chest X-rays, CT scans, and patient symptoms. Additionally, a multimodal approach improved prediction accuracy for patient deterioration by 20%, while reducing hospital stays by 15%. These innovations address inefficiencies and human errors, making diagnostics more reliable and accessible, especially in underserved regions.
Key Takeaways
- Multimodal AI tools use different data, like pictures and records, to make diagnoses more accurate and reliable.
- These tools lower mistakes by studying many data types at once, helping patients get better care.
- AI makes healthcare easier to reach, especially in poor areas, by allowing doctors to check patients remotely and act quickly.
- Teaching doctors how to use AI tools is important so they can understand the results and help patients better.
- Fixing problems like unfairness and keeping data safe is key to making people trust AI and have fair healthcare.
Multimodal AI Agents in Healthcare Diagnostics
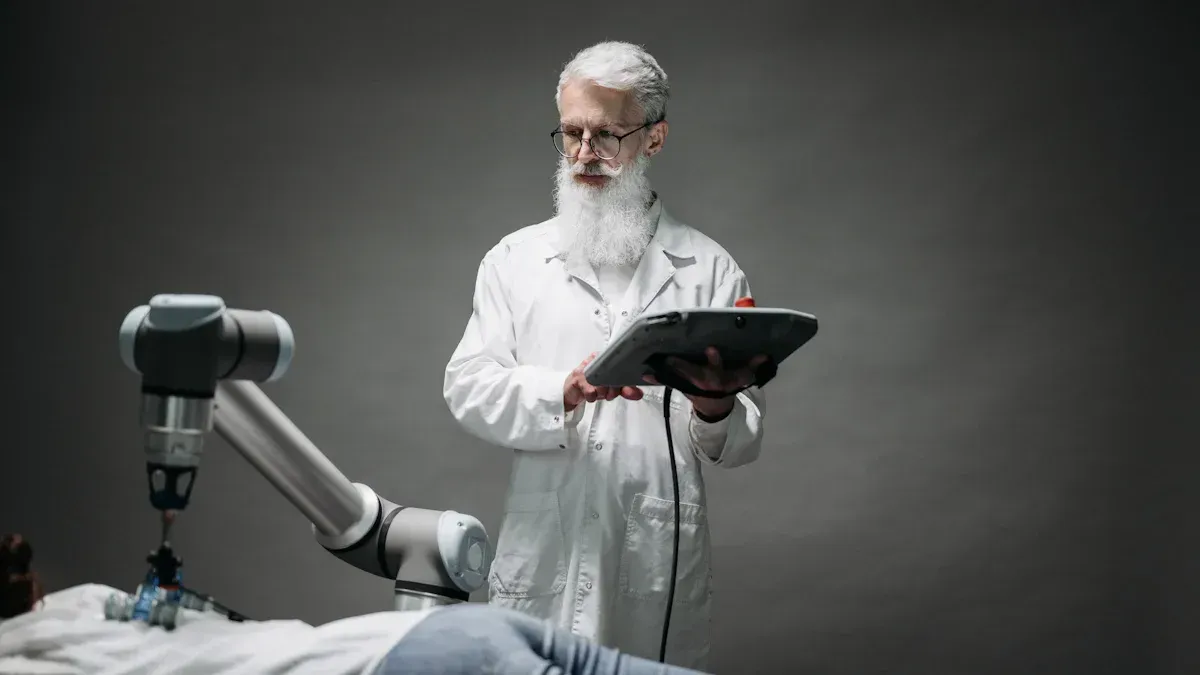
What Are Multimodal AI Agents?
Definition and Key Characteristics of Multimodal AI
Multimodal AI agents combine information from different types of data to create a more complete understanding of complex problems. In healthcare, these agents analyze data like medical images, electronic health records (EHRs), and lab results to provide accurate insights. They excel at integrating structured data, such as numerical lab results, with unstructured data, like doctors' notes or imaging scans.
Multimodal AI stands out because it mimics how humans process information from multiple senses. For example, just as you use both sight and hearing to understand your surroundings, these systems use diverse data sources to improve decision-making.Some key characteristics of multimodal AI include:
- The ability to integrate various data types for better understanding and interaction.
- A focus on improving outcomes, such as diagnosing conditions or predicting patient deterioration.
- Transparency challenges, as complex models can make it harder for users to trust the system.
How Multimodal AI Differs from Traditional AI Systems
Traditional AI systems often rely on a single type of data, like text or images, to perform tasks. In contrast, multimodal AI agents combine multiple data types to deliver more comprehensive results. For instance, while a traditional AI might analyze a chest X-ray, a multimodal AI agent could also consider the patient’s symptoms and medical history to provide a more accurate diagnosis. This holistic approach makes multimodal AI agents invaluable in healthcare diagnostics.
Why Multimodal AI Is Critical in Healthcare
The Importance of Integrating Diverse Data Types for Diagnostics
Healthcare diagnostics often require you to consider multiple factors. Multimodal AI agents simplify this process by connecting data from various sources. This integration enhances predictive accuracy and personalizes care, leading to quicker and more reliable diagnoses. For example, combining imaging data with genomic information can help identify genetic markers for diseases like cancer.
Examples of Healthcare Data Used in Multimodal AI
Multimodal AI agents in healthcare diagnostics utilize a wide range of data, including:
- Medical imaging: X-rays, MRIs, and CT scans.
- Electronic health records (EHRs): Patient histories, prescriptions, and lab results.
- Genomic data: DNA sequences that reveal genetic predispositions.
- Text notes: Doctors’ observations and clinical notes.
The Holistic AI in Medicine (HAIM) framework demonstrates the power of this approach. By integrating tabular data, time-series data, text notes, and imaging, it improved diagnostic accuracy for conditions like pneumonia and lung cancer by 33%. This highlights the clinical importance of using diverse data types in diagnostics.
Core Technologies Driving Multimodal AI in Healthcare
Data Fusion and Integration
Techniques for Harmonizing Structured and Unstructured Data
In healthcare, data comes in many forms, such as numerical lab results, imaging scans, and doctors' notes. Multimodal AI agents excel at combining these structured and unstructured data types to create a unified picture. Techniques like natural language processing (NLP) help extract meaningful insights from text-based records, while computer vision processes imaging data. Advanced algorithms then harmonize these diverse inputs, enabling the system to analyze them together. For example, integrating medical images with patient records and sensor data has significantly improved diagnostic accuracy.
A collaboration between Stanford University and UST demonstrated this by using IoT sensors, audio, images, and video to analyze patient reactions to trauma. This approach highlighted how data fusion can enhance patient outcomes by providing a more comprehensive understanding of their condition.
Overcoming Challenges in Data Standardization
Standardizing healthcare data remains a challenge due to variations in formats and sources. Multimodal AI addresses this by using data preprocessing techniques to clean and organize information. For instance, it can convert handwritten notes into digital text or align imaging data with patient records. These steps ensure that the data is compatible and ready for analysis. By overcoming these hurdles, multimodal AI agents in healthcare diagnostics can deliver more reliable results.
AI Agent Collaboration and Prompt Engineering
How AI Agents Collaborate to Analyze Complex Medical Cases
Multimodal AI agents often work together to solve complex medical problems. Each agent specializes in a specific task, such as analyzing imaging data or interpreting lab results. They then share their findings to create a comprehensive diagnosis. This collaborative approach mimics how medical teams operate, combining expertise from different areas to improve patient care. For example, AI agents analyzing EHR, vital signs, and imaging data have improved patient deterioration prediction accuracy by 20%.
The Role of Natural Language Processing in Interpreting Medical Data
NLP plays a crucial role in helping AI agents understand and interpret unstructured data, such as clinical notes or patient histories. By converting text into structured information, NLP enables the system to identify patterns and correlations that might otherwise go unnoticed. This capability is essential for analyzing complex cases where textual data provides critical context.
Advances in Machine Learning
Neural Networks for Multimodal Data Processing
Neural networks form the backbone of multimodal AI systems. These advanced models process and analyze data from multiple sources simultaneously. For example, convolutional neural networks (CNNs) handle imaging data, while recurrent neural networks (RNNs) focus on sequential data like patient histories. Together, they enable the system to draw insights from diverse inputs, enhancing diagnostic accuracy.
The Impact of Pre-Trained Models and Transfer Learning
Pre-trained models and transfer learning have revolutionized multimodal AI. Pre-trained models, developed using vast datasets, provide a strong foundation for analyzing healthcare data. Transfer learning allows these models to adapt to specific tasks, such as diagnosing chest pathologies. Studies show that using multimodal clinical databases has increased diagnostic accuracy for conditions like pneumonia and lung cancer by 33%. These advancements make multimodal AI agents in healthcare diagnostics more effective and accessible.
Real-World Applications of Multimodal AI Agents in Healthcare Diagnostics
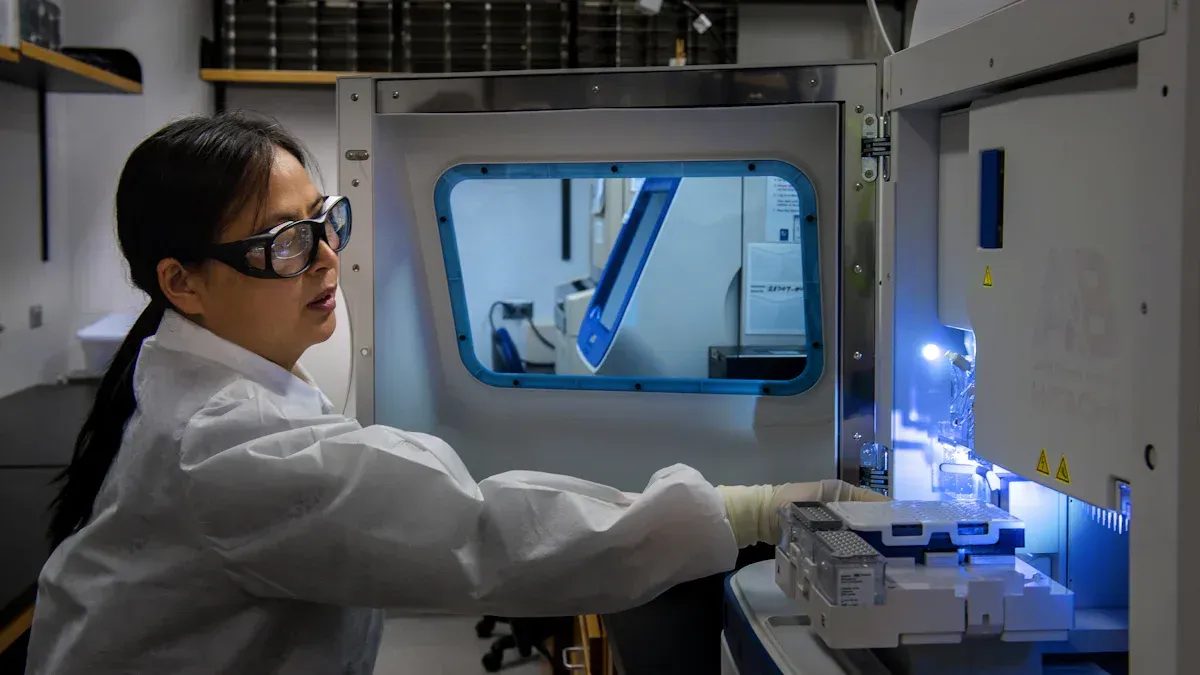
Enhancing Diagnostic Accuracy
AI Tools for Identifying Patterns in Medical Data
AI tools excel at identifying patterns in complex medical data, which improves diagnostic precision. For instance:
- During the COVID-19 pandemic, AI systems combined chest X-rays, CT scans, and patient symptoms to achieve over 90% diagnostic accuracy.
- In MRI analysis, deep learning algorithms assist radiologists by highlighting abnormalities and providing preliminary assessments. This reduces the risk of oversight and enhances diagnostic reliability.
These tools allow you to uncover insights that might be missed through traditional methods, ensuring more accurate and timely diagnoses.
Reducing Diagnostic Errors and Improving Reliability
By integrating diverse data types, multimodal AI agents reduce diagnostic errors significantly. They analyze medical images, patient histories, and lab results simultaneously, offering a holistic view of a patient’s condition. This approach minimizes human error and ensures consistent results. For example, AI systems have demonstrated a 20% improvement in predicting patient deterioration, which helps healthcare providers intervene earlier and more effectively.
Multidisciplinary Diagnosis
Simulating Input from Multiple Specialists for Holistic Analysis
Multimodal AI agents simulate the expertise of multiple specialists, such as cardiologists and neurologists, to deliver a comprehensive diagnosis. These systems analyze medical reports from various perspectives and aggregate the findings into a unified conclusion. This process mirrors how a team of doctors collaborates, ensuring that no critical detail is overlooked. By doing so, you receive a diagnosis that reflects a multidisciplinary approach, enhancing the overall quality of care.
Examples of AI Systems Diagnosing Complex Conditions
AI-powered systems have proven effective in diagnosing complex conditions like cancer and cardiovascular diseases. For example, an AI system can combine imaging data with genomic information to identify genetic markers for cancer. This capability enables early detection and personalized treatment plans, which improve patient outcomes.
Improving Accessibility and Efficiency
AI-Driven Tools for Underserved Regions and Telemedicine
AI-driven diagnostic tools play a crucial role in underserved regions where access to healthcare is limited. A report highlights that approximately 3.6 billion people lack essential health services. AI-powered solutions, combined with 5G technologies, bridge this gap by enabling telemedicine, remote patient monitoring, and predictive analytics. These tools reduce analysis times by 25% and achieve up to 97% accuracy, making timely medical interventions possible even in remote areas.
Accelerating Diagnostic Processes for Timely Treatment
Multimodal AI agents streamline diagnostic workflows, allowing healthcare providers to deliver faster results. By integrating data from multiple sources, these systems reduce the time needed for analysis and decision-making. This acceleration ensures that you receive timely treatment, which is critical for conditions requiring urgent care, such as strokes or heart attacks.
Challenges and Ethical Considerations
Addressing Bias in AI Models
Risks of Biased Training Data and Unequal Outcomes
Bias in AI models can lead to unequal outcomes in healthcare diagnostics. When training data lacks diversity, AI systems may perform poorly for certain patient groups. For example, algorithms trained on data from one region might fail to predict conditions accurately in another due to differences in demographics or treatment practices. This issue has already surfaced in healthcare, where predictive models for breast cancer risk have shown performance gaps, incorrectly assigning black patients as "low risk" more often than others.
Bias can emerge at multiple stages, including data collection, model development, and deployment. It often stems from the system relying on unreliable or incomplete data, which skews predictions and impacts patient care.Strategies to Mitigate Bias in Multimodal AI Systems
You can address bias by implementing strategies like pre-processing data to ensure diversity, in-processing to balance predictions mathematically, and post-processing to refine outputs. Initiatives like TWIX, developed by Kiyasseh et al., offer innovative solutions. TWIX teaches AI models to focus on reliable data sources, reducing bias in predictions. Similarly, programs like STANDING Together emphasize inclusivity in data collection, ensuring AI systems represent diverse populations.
Challenge/Ethical Consideration | Description |
---|---|
Data Acquisition | Gathering diverse datasets can be time-consuming and costly, impacting model performance. |
Fairness | Continuous monitoring is needed to ensure AI systems do not perpetuate biases. |
Transparency | Complex models can lead to mistrust due to opaque decision-making processes. |
Ensuring Data Privacy and Security
Compliance with Regulations Like HIPAA
Data privacy is critical when using AI in healthcare. Regulations like HIPAA require you to protect patient information and prevent unauthorized access. AI systems must comply with these standards to maintain trust and avoid legal repercussions. Organizations can achieve this by establishing internal policies that align with privacy protocols, ensuring secure data handling and storage.
Balancing Data Sharing with Patient Confidentiality
AI systems rely on large datasets to improve accuracy, but sharing data raises privacy concerns. Crowdsourced data, while valuable, risks exposing sensitive information. You can balance this by anonymizing patient data before sharing it or using secure platforms for collaboration. Transparent practices foster trust between patients and healthcare providers, ensuring ethical use of AI in diagnostics.
Integration with Healthcare Systems
Challenges in Adopting AI Within Existing Workflows
Integrating AI into healthcare workflows presents challenges. Existing systems may lack the infrastructure to support advanced AI tools, and adapting them can be costly. Additionally, healthcare professionals may resist change due to unfamiliarity with AI technologies. Overcoming these barriers requires you to invest in training programs and infrastructure upgrades.
Training Healthcare Professionals to Work with AI Tools
Healthcare professionals play a vital role in AI adoption. Training programs can help them understand how AI systems work and how to interpret their outputs. For example, workshops on natural language processing or data fusion techniques can empower you to use AI tools effectively. This collaboration ensures smoother integration and maximizes the benefits of multimodal AI in diagnostics.
Multimodal AI agents are reshaping healthcare diagnostics by combining diverse data types. This integration allows you to benefit from more accurate, efficient, and accessible diagnostic tools. These systems reduce errors, improve reliability, and expand access to underserved areas.
While challenges like bias, privacy concerns, and system integration persist, the potential for better patient outcomes is undeniable.Future research should focus on refining AI models and addressing ethical concerns. Collaboration between AI developers and healthcare professionals will help maximize the impact of these technologies, ensuring they meet your needs effectively.
FAQ
What makes multimodal AI different from traditional AI in healthcare?
s Multimodal AI combines multiple data types, like images and text, to provide a complete analysis. Traditional AI focuses on one data type at a time. This integration allows you to get more accurate and holistic diagnostic results.
Can multimodal AI replace doctors in diagnostics?
No, multimodal AI supports doctors by analyzing data and identifying patterns. It enhances decision-making but doesn’t replace human expertise. You still need doctors to interpret results and provide personalized care.
How does multimodal AI improve healthcare accessibility?
Multimodal AI powers telemedicine and remote diagnostics. It helps underserved regions by analyzing data quickly and accurately. This ensures you receive timely care, even in areas with limited healthcare resources.
Is patient data safe when using multimodal AI systems?
Yes, most systems comply with privacy regulations like HIPAA. They anonymize data and use secure platforms to protect patient information. You can trust these systems to handle your data responsibly.
What challenges do multimodal AI systems face in healthcare?
Challenges include data standardization, bias in models, and integration with existing workflows. Overcoming these requires diverse datasets, ethical practices, and training for healthcare professionals. These steps ensure you benefit from reliable and fair AI tools.
Previous Blogs
How RAG Improves Customer Service Efficiency and Accuracy
AG-based customer service boosts efficiency and accuracy by combining real-time data retrieval with AI, ensuring precise, context-aware responses for customers.
A Comprehensive Guide to Enterprise RAG Implementation Success
Enterprise RAG implementation guide: Avoid pitfalls in self-development, analyze top frameworks, and configure systems for scalability and success.