Generative AI Versus Predictive AI Explained
Artificial intelligence has transformed how you interact with technology. Generative AI and predictive AI represent two powerful branches of this innovation. Generative AI focuses on creating new content by learning from existing data. For example, it can design product features or generate realistic images and music. Predictive AI, on the other hand, analyzes historical data to forecast outcomes. It helps businesses predict consumer demand or identify market trends.
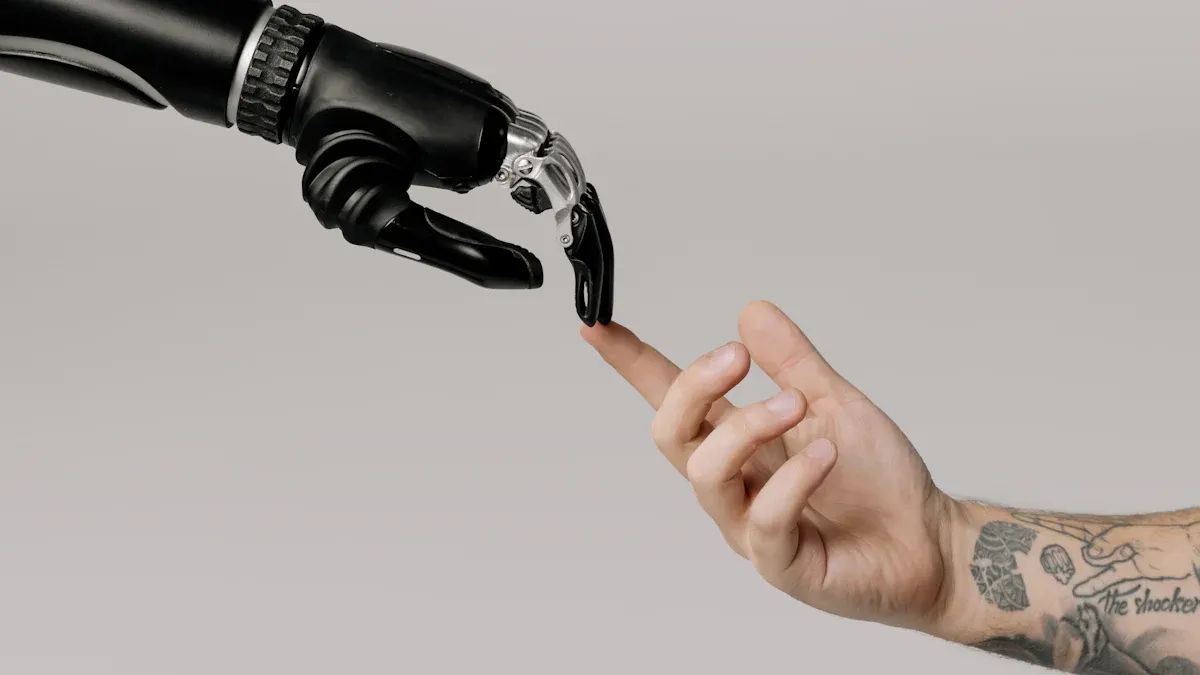
The difference between generative AI and predictive AI lies in their purpose. While generative AI excels at innovation, predictive AI enhances decision-making. Selecting between predictive and generative AI depends on your goals. Understanding what is gen AI vs predictive AI enables you to harness their potential effectively.
Key Takeaways
- Generative AI makes new things like stories and pictures. Predictive AI studies old data to guess future patterns.
- Knowing how generative and predictive AI differ helps you pick the right tool for your needs.
- Both types of AI boost creativity and help with decisions. They are useful in many fields.
- Generative AI saves time by making content automatically. Predictive AI helps companies make smart choices.
- Be careful with AI. Use it responsibly to avoid unfairness and keep people's information safe.
Understanding Artificial Intelligence
What is Artificial Intelligence
Artificial intelligence (AI) refers to the ability of machines to perform tasks that typically require human intelligence. These tasks include learning, reasoning, problem-solving, and decision-making. AI systems analyze data, identify patterns, and make predictions or generate outputs based on their findings. For example, AI can recommend movies based on your viewing history or create realistic images from text descriptions.
AI can be understood in different ways. Some define it broadly as algorithms, which include many non-AI activities. Others describe it as the imitation of human intelligence, though this may not apply to all AI technologies. A more specific definition comes from the European Commission, which states that AI systems analyze their environment and act autonomously to achieve goals. This task-based approach highlights the practical applications of AI in modern technology.
Definition Type | Description |
---|---|
Broad Definition | AI is equated with algorithms, including many non-AI activities. |
Strict Definition | AI imitates human intelligence, though not all technologies fit this view. |
Common Definition | AI enables machines to imitate complex human skills but lacks specificity. |
Task-Based Definition | AI systems analyze environments and act autonomously to achieve goals. |
The Evolution of AI: From Prediction to Generation
AI has evolved significantly over the years. Early AI systems focused on predictive tasks, using historical data to forecast outcomes. These systems helped businesses make decisions by identifying trends and patterns. For instance, predictive AI could analyze sales data to forecast future demand.
Generative AI represents the next stage in this evolution. It goes beyond prediction by creating new content, such as text, images, or music. This shift began with basic models and has advanced to techniques capable of producing original outputs across various fields. Generative AI requires large datasets and diverse models to generate creative content, while predictive AI relies on machine learning to make accurate forecasts.
- Predictive AI focuses on analyzing existing data to make decisions.
- Generative AI creates new content, enhancing creativity and innovation.
- Both types of AI have transformed industries by addressing different needs.
Why Different Types of AI Matter
AI plays a crucial role in modern technology. It enhances operational efficiency, creates new revenue streams, and improves decision-making across industries. For example, AI aids in early detection and treatment of diseases like cancer. It also simplifies complex tasks, such as analyzing evidence in legal investigations, by automating processes and forming concrete hypotheses.
The global AI market reflects its growing importance. The market value exceeds $390 billion and is projected to grow over five times in the next five years. By 2026, the U.S. AI market alone is expected to reach $299.64 billion. This rapid growth highlights the significance of understanding and leveraging different types of AI.
Statistic | Value |
---|---|
Global AI market value | Over $390 billion |
Projected increase in AI industry value | Over 5x in the next 5 years |
Forecast for US AI market by 2026 | $299.64 billion |
CAGR of AI market (2022-2030) | 37.3% |
Expected workforce in AI by 2025 | 97 million |
Companies prioritizing AI in business plans | 83% |
By understanding the differences between generative and predictive AI, you can better appreciate their unique contributions to technology and society.
What is Generative AI
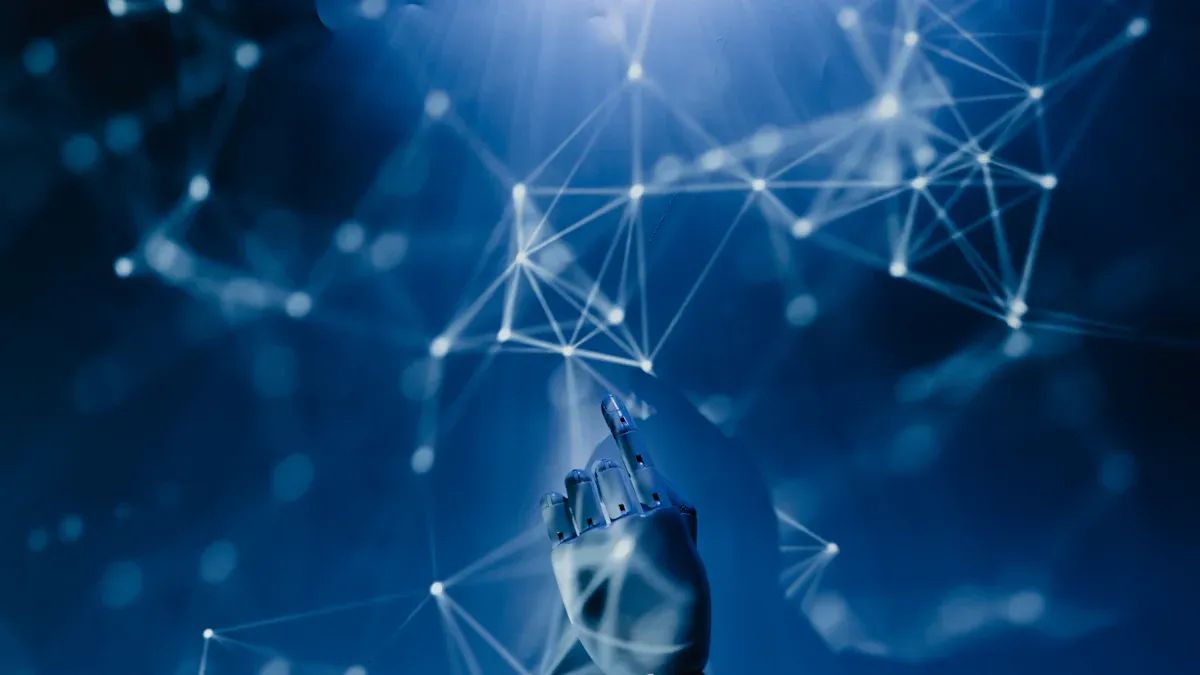
Generative AI refers to artificial intelligence systems that create new content by learning from existing data. Unlike predictive AI, which focuses on forecasting outcomes, generative AI produces original outputs such as text, images, music, or even code. This technology has revolutionized industries by enabling machines to mimic human creativity.
How Generative AI Works
Generative AI relies on advanced machine learning models, particularly neural networks, to generate content. These models analyze vast datasets to identify patterns and relationships. Once trained, they use this knowledge to produce outputs that resemble the original data.
For example, tools like ChatGPT use large language models to generate human-like text. These models evaluate prompts and create responses based on learned patterns. The performance of generative AI is measured using metrics such as fidelity, task performance, and inference speed.
Metric Type | Description |
---|---|
Fidelity | Assesses the similarity between generated output and real data, indicating the model's realism. |
Task Performance | Evaluates how well the model responds to prompts using benchmark datasets. |
Safety Metrics | Tests for ethical concerns, truthfulness, and security risks in generated outputs. |
Inference Speed | Quantifies model performance in terms of speed and efficiency at runtime. |
These metrics ensure that generative AI systems deliver high-quality and reliable results.
Applications of Generative AI
Content creation (e.g., text, images, music)
Generative AI excels in content creation. It can write articles, compose music, and design visuals. For instance, ChatGPT generates emails, while tools like DALL-E create stunning images from text descriptions. This capability has transformed industries like marketing and entertainment.
Virtual assistants and chatbots
Generative AI powers virtual assistants and chatbots, making them more conversational and efficient. These systems can handle customer queries, provide recommendations, and even automate tasks like scheduling. You might have interacted with AI chatbots that feel almost human.
Design and prototyping
In design, generative AI accelerates prototyping by creating multiple iterations quickly. It helps architects, engineers, and product designers visualize concepts. For example, AI-generated UML diagrams simplify software development processes.
Examples of Generative AI Tools
Generative AI tools are widely used across industries:
- Buglistener: Automatically generates bug reports from live chats.
- LLaMA-Reviewer: Automates code review comments using large language models.
- ChatGPT: Writes emails, generates source code, and creates test cases.
- DALL-E: Produces high-quality images from textual prompts.
- Insilico Medicine: Develops new drugs in record time, revolutionizing healthcare.
These tools demonstrate the versatility and impact of generative AI in real-world applications.
What is Predictive AI
Predictive AI uses statistical analysis and machine learning to forecast future events based on historical data. It identifies patterns and trends, enabling you to make informed decisions. This type of AI is widely used across industries to improve efficiency, reduce risks, and enhance customer experiences.
How Predictive AI Works
Predictive AI relies on data-driven processes to generate accurate forecasts. It begins by gathering and cleaning data to ensure quality. The data is then split into training and testing sets. Machine learning algorithms analyze the training data to identify patterns and relationships. These models are tested for accuracy using the testing set before deployment.
Several performance metrics evaluate predictive AI models:
Performance Metric | Description |
---|---|
Error Performance | Measures the accuracy of predictions made by the model. |
Regression | Assesses the relationship between predicted and actual values. |
Error Histogram | Visualizes prediction errors to identify inconsistencies. |
Confusion Matrix | Summarizes the performance of classification models. |
Area under ROC Curve | Evaluates the model's ability to distinguish between different outcomes. |
The success of predictive AI depends on the quality and quantity of training data. High-quality data ensures reliable predictions, while diverse datasets improve the model's adaptability.
Applications of Predictive AI
Forecasting trends (e.g., sales, weather)
Predictive AI excels in trend forecasting. It helps businesses predict sales, optimize inventory, and plan marketing strategies. In weather forecasting, it analyzes historical climate data to provide accurate predictions, aiding industries like agriculture and logistics.
Risk assessment and fraud detection
In finance, predictive AI enhances risk assessment by analyzing credit histories and market trends. It also detects fraudulent activities by identifying unusual patterns in transactions. For example, banks use predictive AI to flag suspicious activities in real time, protecting customers and assets.
Personalized recommendations
Predictive AI powers personalized recommendations in e-commerce, streaming platforms, and online services. It analyzes user behavior to suggest products, movies, or music tailored to your preferences. This improves user satisfaction and boosts engagement.
Sector | Application Description |
---|---|
Healthcare | Improves disease detection, readmission risk prediction, and fraud detection. |
Retail | Enhances inventory management, supply chain efficiency, and dynamic pricing strategies. |
Finance | Supports fraud detection, credit risk assessment, and investment strategy optimization. |
Manufacturing | Enables predictive maintenance, quality control, and resource management. |
Examples of Predictive AI Tools
Predictive AI tools are transforming industries:
- In healthcare, predictive analytics identifies high-risk patients, reducing hospital readmissions and improving care.
- In finance, tools like Zest AI and Socure analyze vast datasets to enhance credit risk assessment and fraud detection.
- In retail, predictive analytics helps businesses understand consumer behavior, enabling tailored marketing strategies.
These tools demonstrate the versatility of predictive AI in solving real-world challenges.
What is Gen AI vs Predictive AI: Key Differences and Similarities
Purpose and Functionality
The difference between generative AI and predictive AI lies in their core objectives. Generative AI focuses on creating new content, such as text, images, or music, by learning from existing data. Predictive AI, however, analyzes historical data to forecast future outcomes. For example, generative AI can design unique marketing visuals, while predictive AI helps businesses anticipate sales trends.
Aspect | Generative AI | Predictive AI |
---|---|---|
Purpose | Creates new data similar to training data | Analyzes historical data to predict future outcomes |
Input and Output | Requires prompts to generate novel content | Uses historical data to make forecasts |
Model Architecture | Utilizes GANs and neural networks | Employs statistical algorithms and machine learning |
Generative AI enhances creativity by producing novel outputs. Predictive AI excels in decision-making by identifying patterns in past data. This distinction is crucial when selecting the right technology for your needs.
Data Requirements and Processing
Generative AI and predictive AI differ significantly in their data requirements and processing approaches. Generative AI relies on unstructured data, such as images or text, and requires intensive computational resources. Predictive AI, on the other hand, uses structured historical data and has lighter computational needs.
Aspect | Generative AI | Predictive AI |
---|---|---|
Data Type | Unstructured data | Structured historical data |
Purpose | Creates new content | Forecasts future events |
Computational Needs | Intensive computational requirements | Lighter computational needs |
Deployment Speed | Longer setup and training period | Faster deployment for specific tasks |
Generative AI often takes longer to train and deploy due to its complexity. Predictive AI, with its simpler models, offers quicker implementation for specific tasks like fraud detection or inventory management.
Applications and Use Cases
Both generative AI and predictive AI have transformative applications across industries. Generative AI powers creative media, such as art and music, and supports data augmentation for training models. Predictive AI drives market forecasting, healthcare diagnostics, and fraud detection.
- Generative AI Applications:
- Creative media (e.g., designing visuals, composing music)
- Data augmentation for improving machine learning models
- Predictive AI Applications:
- Market forecasting to predict sales trends
- Healthcare diagnostics for early disease detection
- Fraud detection, saving banks up to $16 million annually
Generative AI could add between $2.6 trillion to $4.4 trillion annually across various sectors. Meanwhile, the predictive AI market is projected to reach $64 billion by 2025. These figures highlight the immense potential of both technologies in shaping the future.
Similarities Between Generative and Predictive AI
Generative AI and predictive AI may serve different purposes, but they share several foundational similarities. Understanding these commonalities helps you see how both technologies complement each other in advancing artificial intelligence.
1. Data-Driven Foundations
Both generative and predictive AI rely heavily on data. They process vast amounts of information to identify patterns and extract insights. For example, generative AI uses datasets to create new content, while predictive AI analyzes historical data to forecast outcomes. Without quality data, neither system can function effectively.
2. Machine Learning at Their Core
Both types of AI use machine learning (ML) algorithms to achieve their goals. Generative AI often employs neural networks, such as Generative Adversarial Networks (GANs), to produce outputs. Predictive AI uses statistical models and supervised learning to make accurate predictions. In both cases, ML enables the systems to learn and improve over time.
3. Automation and Efficiency
Generative and predictive AI automate complex tasks, saving you time and resources. Generative AI can create marketing content or design prototypes, while predictive AI streamlines decision-making by forecasting trends. This shared ability to enhance efficiency makes them valuable tools across industries.
Aspect | Predictive AI | Generative AI |
---|---|---|
Data Dependency | Relies on historical data for analysis | Requires large datasets for training |
Learning Approach | Employs supervised learning models | Uses neural networks like GANs |
Outcome | Forecasts future events | Creates new content |
💡 Tip: Combining generative and predictive AI can unlock even greater potential. For instance, predictive AI can identify trends, and generative AI can create tailored content based on those insights.
4. Transformative Impact
Both technologies are reshaping industries. Healthcare, finance, and entertainment benefit from their capabilities. For example, predictive AI improves diagnostics, while generative AI designs new drugs. Together, they drive innovation and solve real-world problems.
By recognizing these similarities, you can better understand how generative and predictive AI work together to revolutionize technology.
Benefits and Limitations of Generative and Predictive AI
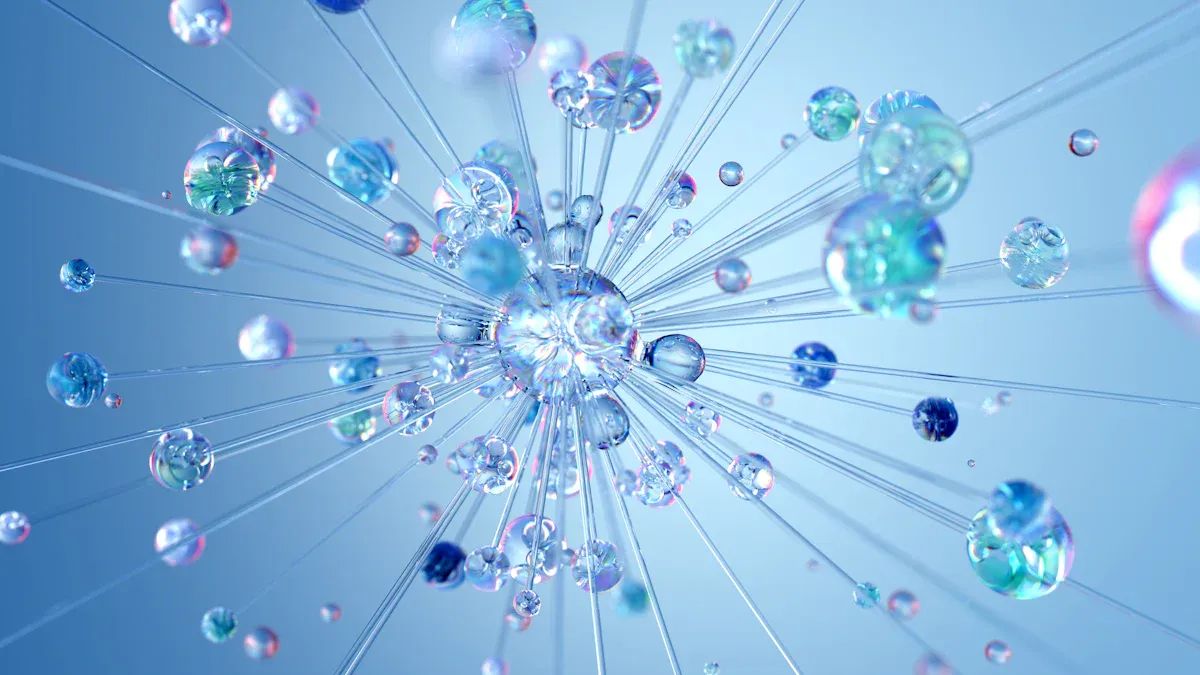
Benefits of Generative AI
Creativity and innovation
Generative AI empowers you to unlock new levels of creativity. By analyzing large datasets, it generates original content such as text, images, and music. This capability has revolutionized industries like marketing, entertainment, and design. For example, tools like DALL-E create stunning visuals from simple text prompts, enabling you to bring innovative ideas to life. Generative AI also supports data augmentation, which enhances machine learning models by creating diverse datasets.
Metric Type | Description |
---|---|
Model Performance | Assesses the effectiveness of generative AI in producing high-quality outputs. |
Innovation Score | Measures the frequency of novel and useful ideas generated by the AI. |
User Satisfaction | Evaluates how well generative AI meets user expectations for creativity and originality. |
These metrics highlight how generative AI drives innovation and delivers value across various sectors.
Automation of content creation
Generative AI simplifies content creation by automating repetitive tasks. It can write emails, generate marketing copy, or even compose music. This automation saves you time and resources, allowing you to focus on strategic goals. For instance, ChatGPT helps businesses draft professional emails in seconds. According to industry estimates, generative AI could add between $2.6 trillion to $4.4 trillion annually across multiple sectors, showcasing its transformative potential.
Benefits of Predictive AI
Improved decision-making
Predictive AI acts as a powerful decision-making tool. By analyzing historical data, it helps you forecast trends, assess risks, and make informed choices. For example, UPS uses predictive AI to optimize delivery routes, saving $35 million annually. This technology enhances your ability to anticipate challenges and seize opportunities, making it invaluable for industries like finance, healthcare, and logistics.
Enhanced efficiency and accuracy
Predictive AI streamlines operations by automating complex analyses. It simplifies data processing, identifies patterns, and delivers accurate forecasts. This efficiency reduces costs and improves productivity. For example, predictive AI excels in demand sensing, inventory optimization, and preventive maintenance. These applications ensure that your business runs smoothly and adapts to changing conditions effectively.
Aspect | Predictive AI | Generative AI |
---|---|---|
Primary Focus | Data analysis and forecasting | Content creation and innovation |
Best Use Cases | Financial forecasting, fraud detection | Customer service, marketing content |
Resource Needs | Lighter computational requirements | Intensive computational requirements |
Limitations of Generative AI
Risk of generating inaccurate or biased content
Generative AI relies on training data, which can introduce biases or inaccuracies into its outputs. For example, AI hallucinations may produce misleading or false information. This lack of explainability raises concerns about data trustworthiness. You must carefully monitor and refine generative AI models to minimize these risks.
High computational requirements
Generative AI demands significant computational power, which can increase costs and limit accessibility. Training large models requires advanced hardware and substantial energy resources. While these challenges may not affect smaller-scale applications, they can pose barriers for businesses with limited budgets.
Note: Balancing the benefits of generative AI with its limitations requires thoughtful implementation and ongoing evaluation.
Limitations of Predictive AI
Dependence on quality and quantity of data
Predictive AI relies heavily on data to function effectively. The quality and quantity of this data directly impact the accuracy of its predictions. When you use predictive AI, poor-quality data can lead to biased results. For example, if the training data contains errors or reflects societal biases, the AI might amplify these issues. This can create inconsistencies in predictions and raise ethical concerns.
Large datasets are essential for predictive AI to identify patterns and trends. However, gathering and maintaining such datasets can be challenging. Incomplete or outdated data reduces the model's reliability. Overfitting is another risk. When a model becomes too tailored to its training data, it struggles to generalize, leading to inaccurate predictions in real-world applications.
Predictive AI also faces challenges with interpretability. Complex models, such as deep learning algorithms, often act as "black boxes." This makes it difficult for you to understand how the AI arrived at a particular prediction. Without transparency, trusting the system's outputs becomes harder.
- Bias in data can distort predictions.
- Overfitting limits the model's adaptability.
- Lack of interpretability complicates decision-making.
Limited ability to handle novel scenarios
Predictive AI excels at analyzing historical data, but it struggles with unfamiliar situations. When you encounter a scenario that deviates from past patterns, the AI may fail to provide accurate predictions. This limitation stems from its reliance on historical data. Overreliance on past trends hinders the model's ability to adapt to new circumstances.
For instance, during unprecedented events like the COVID-19 pandemic, many predictive AI systems failed to forecast market behaviors accurately. These models could not account for the sudden changes in consumer habits or economic conditions. Predictive AI often confuses correlation with causation, further complicating its ability to handle novel scenarios. This can lead to flawed insights and poor decision-making.
To address these challenges, you must combine predictive AI with human expertise. While the AI identifies patterns, humans can interpret the results and apply them to unique situations. This collaboration ensures better outcomes, especially in dynamic environments.
Note: Predictive AI is a powerful tool, but its limitations highlight the importance of high-quality data and human oversight.
Ethical Considerations for Generative and Predictive AI
Ethical Concerns in Generative AI
Deepfakes and misinformation
Generative AI has the power to create highly realistic content, but this capability comes with risks. Deepfake videos and fabricated news articles can manipulate public opinion and spread misinformation. For example, AI-generated deepfakes have been used to impersonate public figures, eroding trust in media. These tools can also produce harmful content, which may damage brand integrity or mislead consumers. AI chatbots have even misrepresented corporate policies, highlighting the dangers of misinformation in automated systems.
To address these issues, you must implement strict content monitoring and verification processes. Companies should also educate users about the potential risks of generative AI outputs to build awareness and trust.
Intellectual property issues
Generative AI often uses vast datasets for training, which can lead to copyright and legal challenges. For instance, AI-generated content might unknowingly replicate copyrighted material, exposing companies to legal risks. The unknown sources of generated outputs can also create reputational risks, especially in industries like finance or healthcare.
To mitigate these concerns, you should ensure that your AI systems comply with intellectual property laws. Transparent documentation of training data sources can help reduce legal exposure and maintain ethical standards.
Ethical Concerns in Predictive AI
Bias in data and decision-making
Predictive AI relies on historical data, which can perpetuate existing biases. For example, biased training data has led to unfair evaluations in hiring and promotions. A documented case involved a facial recognition system with higher error rates for people of color, highlighting the need for fairness in AI development.
You can address these issues by curating diverse datasets and implementing fairness constraints. Regular audits of predictive AI models can also help identify and reduce bias, ensuring equitable outcomes.
Privacy and surveillance concerns
Predictive AI processes large volumes of data, raising concerns about privacy and security. Unauthorized access to sensitive information can lead to breaches, compromising user confidentiality. For example, predictive AI in healthcare must handle patient data responsibly to avoid ethical violations.
To safeguard privacy, you should adopt robust data protection measures and comply with regulatory standards. Transparent data usage policies can also reassure users and foster trust in predictive AI systems.
Balancing Innovation with Responsibility
Balancing innovation with ethical responsibility requires a proactive approach. Companies must leverage existing regulatory frameworks while addressing gaps introduced by AI capabilities. Collaboration between industry, academia, and civil society can foster holistic governance. For example, agile regulatory frameworks can prepare you for the rapid evolution of AI technologies.
Pillar | Description |
---|---|
Harness past | Use existing regulations while addressing new AI-related challenges. |
Build present | Collaborate across sectors for comprehensive governance. |
Plan future | Develop flexible policies to adapt to AI advancements. |
By adopting these strategies, you can ensure that generative AI and predictive AI drive innovation responsibly while minimizing ethical risks.
Generative AI and predictive AI are reshaping industries with their unique capabilities. Generative AI drives creativity by producing original content, while predictive AI enhances decision-making through accurate forecasts. Understanding the difference between generative AI and predictive AI helps you choose the right tool for your needs.
You must also consider the ethical implications of these technologies. Responsible development ensures that generative AI and predictive AI benefit society without causing harm. By leveraging their strengths wisely, you can unlock their full potential and stay ahead in a rapidly evolving world.
💡 Tip: Explore tools like PuppyAgent to integrate AI into your workflows and maximize efficiency.FAQ
What is the main difference between generative AI and predictive AI?
Generative AI creates new content, such as text or images, by learning from existing data. Predictive AI analyzes historical data to forecast future outcomes. While generative AI focuses on creativity, predictive AI enhances decision-making through data-driven insights.
Can generative AI and predictive AI work together?
Yes, they can complement each other. Predictive AI identifies trends or patterns, and generative AI uses those insights to create tailored content. For example, predictive AI forecasts customer preferences, and generative AI designs personalized marketing materials.
What industries benefit the most from predictive AI?
Predictive AI is widely used in healthcare, finance, retail, and logistics. It improves decision-making, optimizes operations, and reduces risks. For instance, it helps doctors predict disease risks and assists businesses in forecasting sales trends.
Is generative AI suitable for small businesses?
Yes, many generative AI tools are accessible and cost-effective for small businesses. Platforms like ChatGPT or DALL-E offer free or affordable plans, enabling small teams to automate content creation and enhance creativity without significant investment.
How does PuppyAgent fit into the AI landscape?
PuppyAgent combines the strengths of generative and predictive AI. It uses Retrieval-Augmented Generation (RAG) to process data efficiently and deliver actionable insights. This makes it ideal for businesses looking to streamline workflows and enhance knowledge management.
💡 Tip: Explore PuppyAgent's free plan to see how it can transform your business operations.Previous Blogs
Understanding Manus AI Agent and Its Revolutionary Impact
Manus AI Agent is a game-changing AI that turns your ideas into reality, automating complex tasks across industries like finance and education to boost productivity and deliver real results—discover how it works now!
Analyzing the Power of Generative AI in the Metaverse
Generative AI is transforming the metaverse by enabling dynamic, personalized virtual experiences through advanced content creation and adaptive environments, while presenting challenges related to ethics, bias, and intellectual property.