Effective Strategies for AI Implementation in Smart Manufacturing
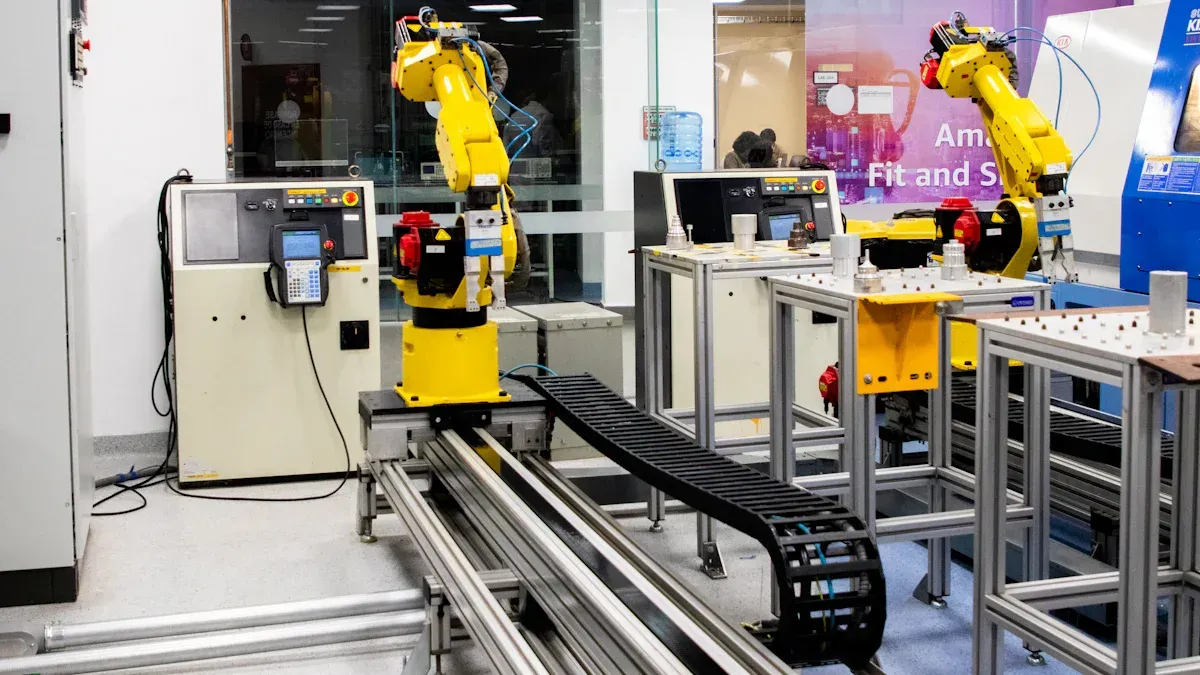
Artificial intelligence is reshaping the manufacturing industry, unlocking new levels of efficiency and innovation. By 2025, AI will contribute up to $15.7 trillion to the global manufacturing sector. It can reduce forecasting errors by 50% and downtime losses by the same margin, ensuring smoother operations. AI-driven systems also achieve up to 90% accuracy in defect detection, improving product quality by as much as 35%. These advancements not only enhance productivity but also create opportunities, with AI expected to generate over 12 million jobs. Embracing AI in Smart Manufacturing: Implementation Strategies empowers you to stay competitive in this rapidly evolving landscape.
Key Takeaways
- AI can greatly improve manufacturing by cutting errors and downtime by half.
- Use AI for predictive maintenance to predict equipment issues, making machines last longer and avoiding sudden breakdowns.
- Use IoT gadgets to gather live data, helping make better choices and improving work.
- Teach your workers about AI tools to help them do more and bring new ideas to your company.
- Begin using AI with small test projects to save money and show clear benefits.
Understanding AI in Smart Manufacturing
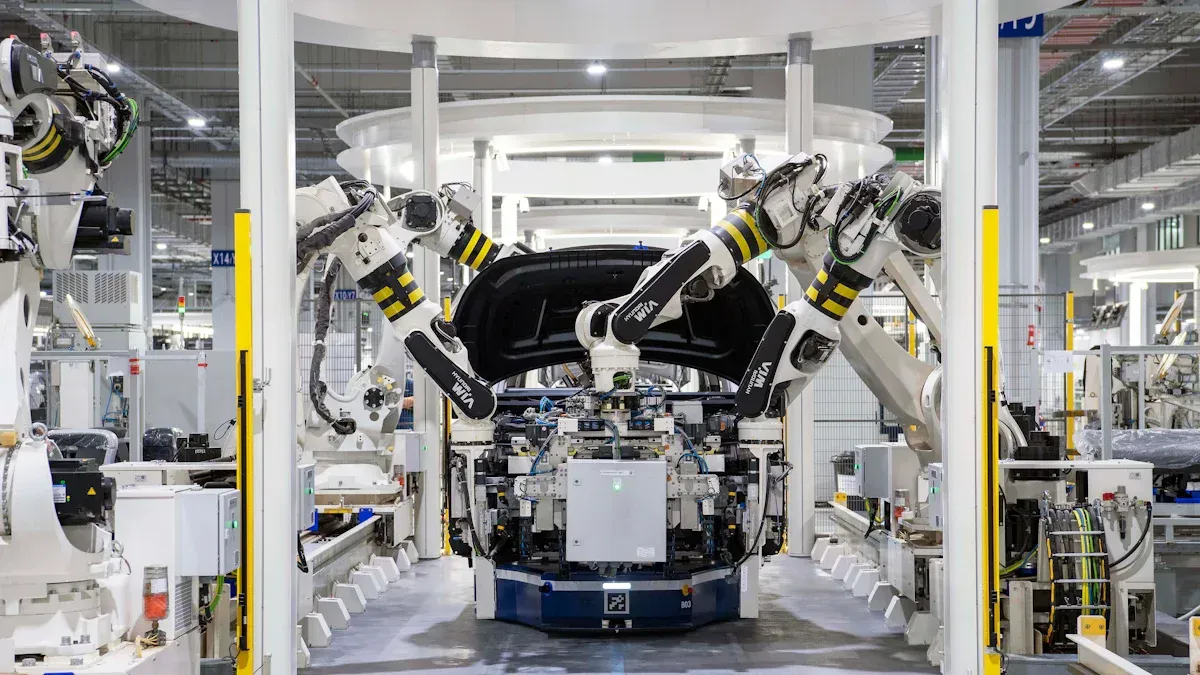
Benefits of AI in Manufacturing
Data-driven decision-making for operational excellence
AI empowers you to make smarter decisions by analyzing vast amounts of data in real time. For example, companies like Honeywell use AI platforms to monitor operations and provide actionable insights. This approach reduces inefficiencies and ensures optimal performance. According to Deloitte, manufacturing generates more data than any other industry, making AI a critical tool for transforming raw data into meaningful strategies.
Predictive maintenance to minimize downtime
AI algorithms predict equipment failures before they occur. This proactive approach reduces downtime and extends the lifespan of machinery. For instance, Honeywell's Forge platform uses predictive analytics to monitor equipment conditions and prevent costly breakdowns. A Deloitte survey revealed that 66% of manufacturers rely on AI to enhance operational reliability.
Enhanced quality control through automated systems
AI vision systems inspect products with remarkable speed and accuracy. These systems detect defects that human inspectors might miss, achieving up to 90% accuracy. This improvement leads to higher-quality products and fewer returns. Companies like Rockwell Automation leverage AI tools to streamline quality control, boosting productivity and customer satisfaction.
The Evolution of AI in Manufacturing
Transitioning from automation to AI-driven processes
Manufacturing has evolved from basic automation in the 1960s to AI-powered systems today. AI now enables machines to learn and adapt, optimizing processes without human intervention. For example, a global electronics manufacturer modernized its production lines with AI, addressing inefficiencies and improving throughput by 20%.
Integrating IoT, robotics, and AI for smarter systems
The integration of IoT, robotics, and AI creates interconnected systems that enhance efficiency. IoT devices collect real-time data, while AI analyzes it to optimize operations. Advanced robotics powered by AI automate complex tasks, reducing errors and increasing productivity. This synergy forms the backbone of smart factories, where digital twins simulate and refine processes before implementation.
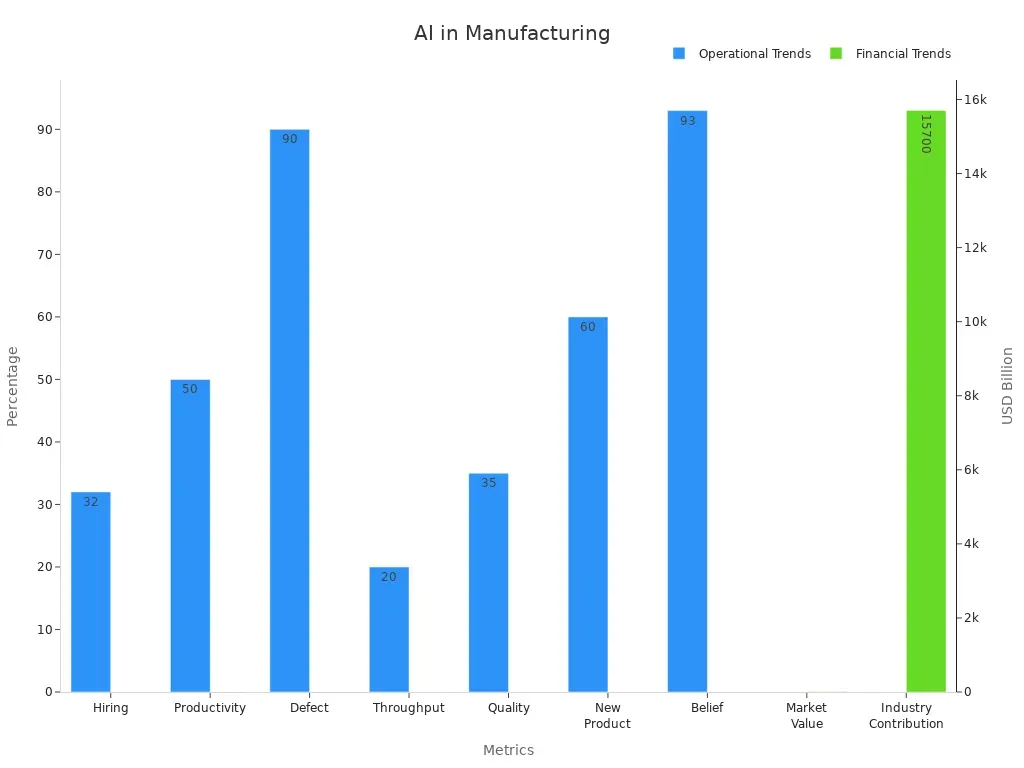
AI in Smart Manufacturing: Implementation Strategies
Leveraging IoT for Real-Time Data
Connecting devices for seamless data collection
IoT devices play a crucial role in gathering real-time data from manufacturing equipment. These sensors monitor parameters like temperature, vibration, and energy usage, providing valuable insights into machine performance. For example, real-time monitoring enables you to detect anomalies early, ensuring smooth operations. Predictive maintenance, powered by IoT, reduces unexpected breakdowns by analyzing sensor data to predict potential failures. This approach enhances equipment uptime by 10-20% and cuts maintenance costs by 5-10%.
Using AI to analyze and act on real-time insights
AI processes the vast amounts of data collected by IoT devices, transforming it into actionable insights. By identifying performance trends and anomalies, AI helps you optimize operations and improve efficiency. For instance, predictive analytics can reduce maintenance planning time by up to 50%. This combination of IoT and AI ensures smarter decision-making and better resource allocation.
Integrating AI into Production and Quality Control
Deploying AI tools for defect detection and correction
AI-powered vision systems revolutionize quality control by detecting defects with up to 90% accuracy. These systems inspect products faster and more precisely than human inspectors, leading to fewer errors and higher-quality outputs. Companies like Intel use machine learning to optimize yields and enhance defect detection, ensuring consistent product quality.
Automating repetitive tasks to boost productivity
AI automates repetitive tasks, freeing up your workforce for more complex responsibilities. This automation improves production throughput by up to 20% and reduces operational costs. For example, robotic systems powered by AI handle assembly line tasks efficiently, minimizing errors and increasing productivity.
Transitioning to Smart Factories
Creating digital twins for simulation and optimization
Digital twins replicate physical systems in a virtual environment, allowing you to simulate and optimize processes before implementation. General Electric uses digital twins to predict equipment performance, reducing unexpected downtime by 40% and cutting upkeep expenses by 20%. This technology enhances operational efficiency and scalability.
Implementing AI-driven supply chain management
AI streamlines supply chain operations by analyzing data to forecast demand, optimize inventory, and reduce costs. Toyota, for instance, uses AI to manage its supply chain, achieving a 20% reduction in inventory costs and a 15% decrease in energy consumption. These improvements ensure a more agile and sustainable manufacturing process.
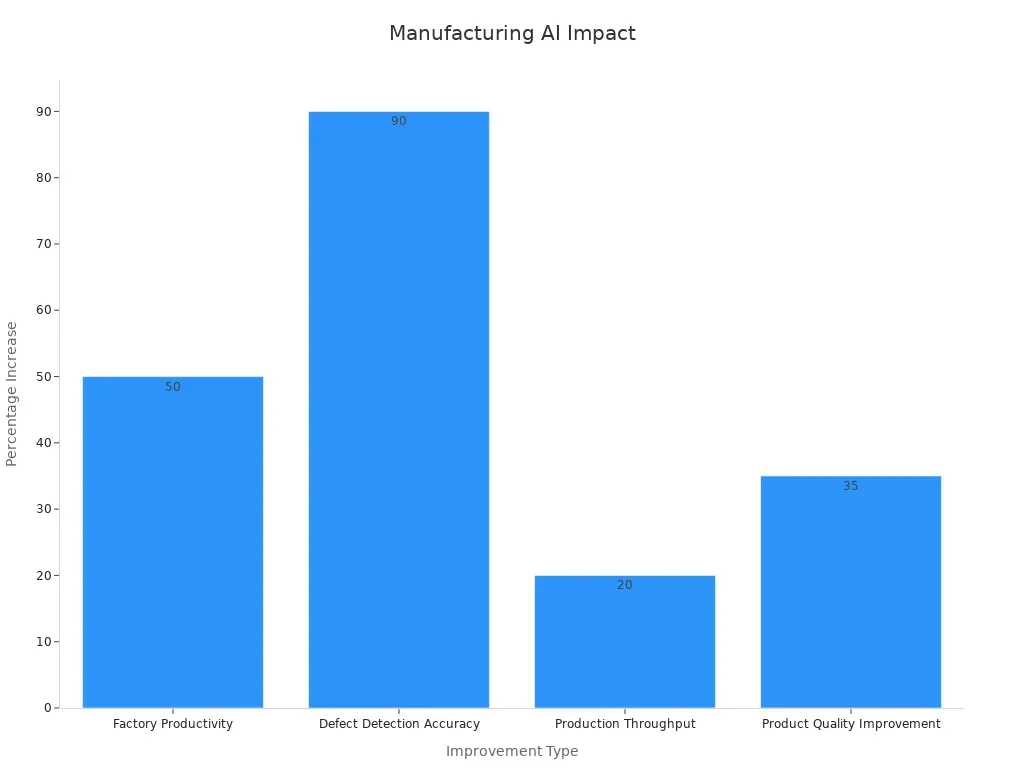
Actionable Steps for Implementation
Conducting readiness assessments for AI adoption
Before implementing AI, you need to evaluate your organization's readiness. Start by identifying your goals and aligning them with your business strategy. Assess your current infrastructure, including hardware, software, and data systems. Determine if your existing equipment can support AI technologies or if upgrades are necessary. For example, Siemens conducted a thorough readiness assessment before integrating AI into its manufacturing processes. This step helped them reduce unplanned downtime and improve product consistency. A clear understanding of your starting point ensures a smoother transition to AI-driven systems.
Training employees to work with AI technologies
Your workforce plays a critical role in the success of AI implementation. Equip your employees with the skills needed to operate and manage AI tools. Offer hands-on training programs and workshops to familiarize them with new technologies. Collaborate with educational institutions to develop tailored training modules. For instance, an electronics manufacturer improved operational efficiency by upskilling its employees to work with AI systems. When your team feels confident using AI, they can maximize its potential and drive innovation.
Partnering with AI solution providers for tailored systems
Collaborating with experienced AI solution providers can simplify the implementation process. These providers offer customized solutions that address your specific needs. They also help integrate AI with your existing systems, ensuring seamless operation. For example, many organizations have partnered with AI experts to enhance decision-making and automate processes. This approach allows you to focus on scaling and optimizing your operations while leveraging the expertise of professionals. Building strong partnerships ensures that your AI systems align with your business objectives.
Tip: Follow a step-by-step approach to implement AI effectively. Start with a clear strategy, establish connectivity, integrate systems, develop analytics, and scale gradually. This method has proven successful in smart manufacturing environments.Overcoming Challenges in AI Implementation
Addressing Cost and ROI Concerns
Starting with small-scale, phased implementation
Starting small allows you to manage costs effectively while testing AI's potential. A phased approach minimizes risks and ensures smoother integration into your existing systems. For instance, implementing AI in one production line before scaling across the factory helps you identify challenges early. This strategy also allows you to allocate resources efficiently and avoid unnecessary expenses. Flexible and scalable AI systems adapt to your business needs, ensuring long-term cost-effectiveness.
Demonstrating ROI through pilot projects
Pilot projects provide measurable results, helping you justify AI investments. By focusing on specific areas like defect detection or predictive maintenance, you can showcase tangible benefits such as increased productivity or reduced downtime. For example, phased AI implementations have shown up to a 50% productivity increase and a 90% improvement in defect detection accuracy.
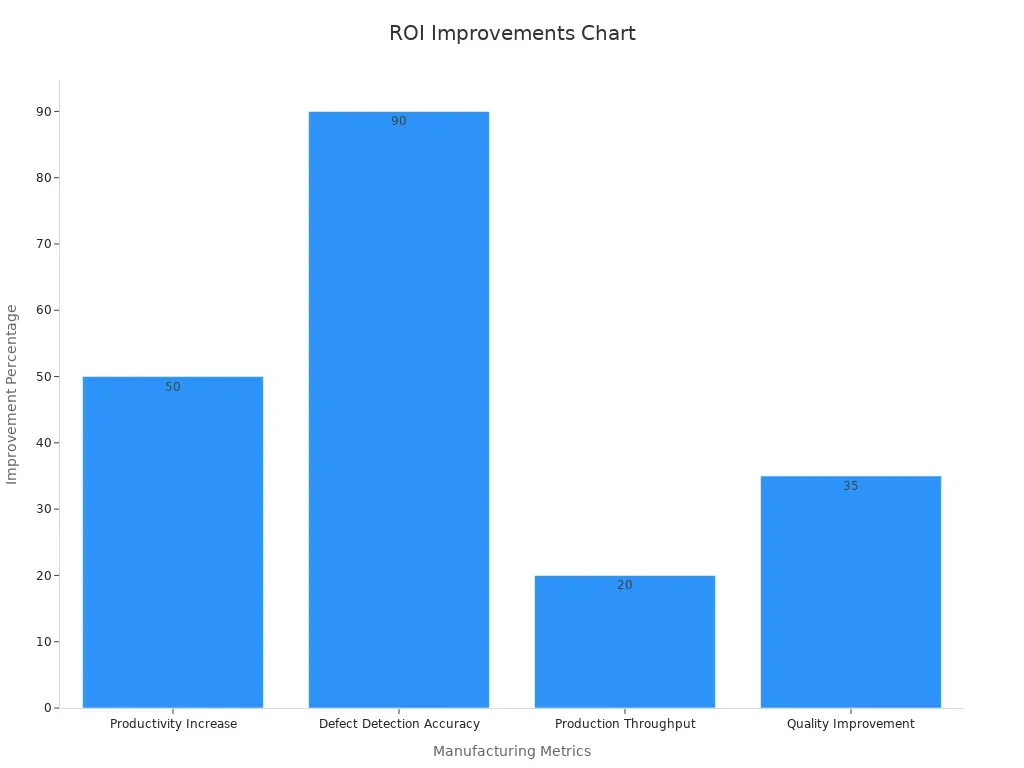
Bridging the Skills Gap
Upskilling employees with AI training programs
Your workforce needs the right skills to operate AI systems effectively. Training programs enhance readiness by developing specific AI competencies. Hands-on workshops and online courses can help employees understand AI tools and their applications. For example, companies investing in training have reported smoother AI adoption and improved operational efficiency.
Collaborating with educational institutions for talent development
Partnering with universities and technical schools ensures a steady pipeline of skilled talent. These collaborations can include internships, research projects, or tailored training modules. By fostering such partnerships, you not only bridge the skills gap but also promote innovation within your organization.
Ensuring Data Integration and Security
Establishing robust data governance frameworks
Effective data management is critical for AI success. Invest in modern data infrastructure, including IoT devices and sensors, to improve data collection and integration. Data governance frameworks ensure that your data remains accurate, consistent, and accessible. This approach reduces integration issues, which are a common hurdle during AI adoption.
Using secure cloud platforms for AI data processing
Data security is a top priority when implementing AI. Secure cloud platforms offer encryption protocols, access controls, and regular security audits to protect sensitive information. Federated learning further enhances security by allowing AI models to train across multiple datasets without sharing raw data. These measures significantly reduce the risk of data breaches, ensuring compliance and trustworthiness.
Future Trends and Measuring Success
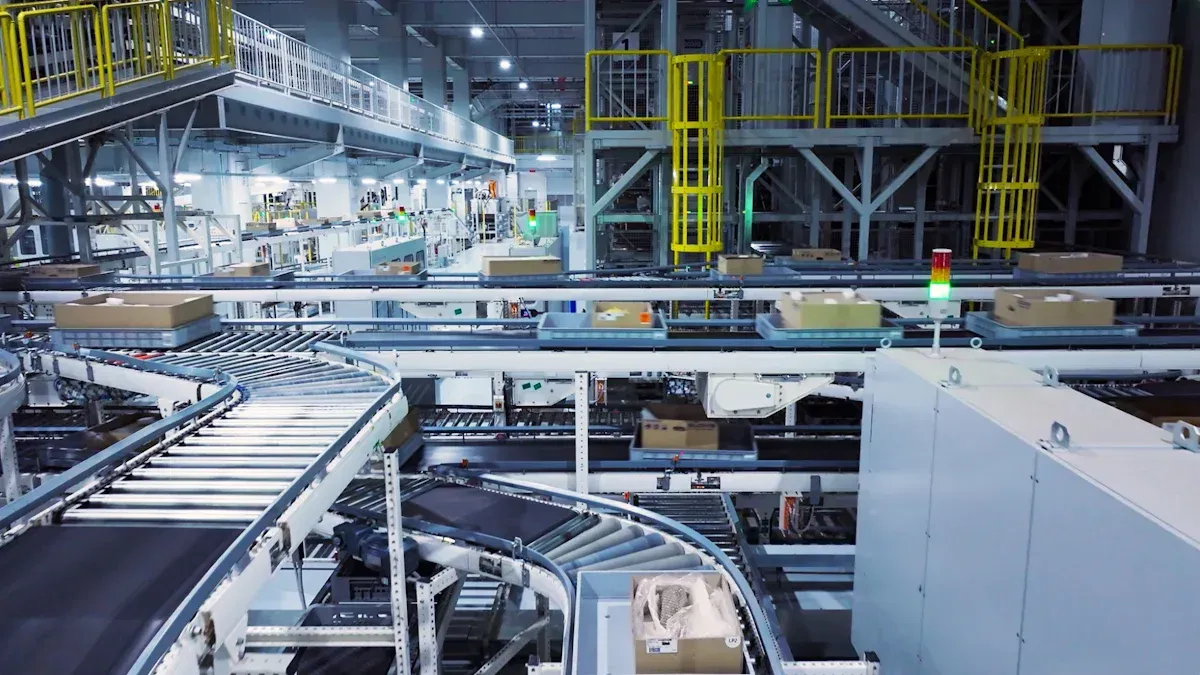
Emerging Technologies in Smart Manufacturing
Generative AI for design and prototyping
Generative AI is transforming how you approach design and prototyping. By analyzing existing designs, it creates innovative solutions that meet specific requirements. This technology accelerates the design process, reducing time-to-market. For example, generative AI can suggest optimal material usage, cutting costs and improving sustainability. It also enables rapid prototyping, allowing you to test multiple iterations virtually before physical production begins.
AI-powered robotics for adaptive manufacturing
AI-powered robotics bring adaptability to your manufacturing processes. These robots learn from data and adjust to changing conditions in real time. For instance, they can handle complex assembly tasks with precision, reducing errors and increasing productivity. Adaptive robotics also improve workplace safety by taking over hazardous tasks. This technology ensures your factory remains efficient and responsive to market demands.
Key Metrics for Success
Monitoring overall equipment effectiveness (OEE)
OEE is a critical metric for evaluating your factory's performance. It measures availability, performance, and quality to provide a comprehensive view of efficiency. World-class factories aim for an OEE score of 85% or higher. For example, the automotive industry typically achieves scores between 65-80%. Tracking OEE helps you identify bottlenecks and optimize operations.
Industry | Average OEE Score |
---|---|
Automotive | 65-80% |
Pharmaceutical | 70-85% |
World-Class Benchmark | 85% or higher |
Tracking energy consumption and sustainability goals
Energy efficiency is vital for sustainable manufacturing. AI helps you monitor energy usage and identify areas for improvement. For example, predictive analytics can optimize energy-intensive processes, reducing costs and environmental impact. Tracking these metrics ensures your factory aligns with sustainability goals while maintaining profitability.
Preparing for the Future
Staying agile to adapt to new AI advancements
Agility is essential for keeping up with AI advancements. Predictive maintenance, quality control, and supply chain optimization are evolving rapidly. By adopting flexible systems, you can integrate new technologies seamlessly. For instance, AI-driven predictive maintenance reduces downtime and costs by analyzing real-time data.
Building a culture of continuous innovation
Fostering innovation ensures your factory stays competitive. Encourage your team to explore new ideas and embrace AI-driven solutions. Collaborate with industry leaders and educational institutions to stay ahead of trends. Companies like Siemens have demonstrated the benefits of continuous innovation, achieving higher productivity and reduced downtime through AI-driven systems.
Tip: Regularly review your AI strategies to ensure they align with your business goals and industry advancements.AI has revolutionized smart manufacturing, delivering remarkable benefits across operations. By 2025, it will contribute $15.7 trillion to the industry, reduce forecasting errors and downtime losses by 50%, and improve product quality by 35%. These advancements highlight the transformative potential of AI when implemented strategically.
Statistic Description | Value |
---|---|
Contribution of AI to manufacturing industry by 2025 | $15.7 trillion |
Reduction in forecasting errors | 50% |
Reduction in downtime losses | 50% |
Accuracy in identifying defects | 90% |
Improvement in product quality | 35% |
Boost in production processes | 10% to 15% |
Increase in EBITA | 4% to 5% |
Savings in repair costs through predictive maintenance | 40% |
Strategic implementation ensures you overcome challenges like cost concerns and skills gaps. By embracing AI, you position your business for sustained growth and innovation. The future belongs to those who adapt and innovate. Will you lead the way?
FAQ
What is the first step to implementing AI in manufacturing?
Start by conducting a readiness assessment. Evaluate your current infrastructure, data systems, and workforce capabilities. Identify specific goals for AI adoption, such as improving quality control or reducing downtime. This step ensures a clear roadmap for successful implementation.
How can AI improve quality control in manufacturing?
AI-powered vision systems detect defects with high accuracy. These systems analyze products faster than human inspectors, ensuring consistent quality. By automating inspections, you reduce errors and improve customer satisfaction. AI tools also provide real-time feedback, helping you address issues immediately.
Is AI implementation expensive for small manufacturers?
AI implementation can start small and scale gradually. Begin with pilot projects to test its effectiveness. Many AI solutions offer flexible pricing models, making them accessible for smaller businesses. This approach minimizes upfront costs while demonstrating measurable returns on investment.
How do you train employees to work with AI technologies?
Offer hands-on training programs and workshops. Partner with educational institutions to create tailored courses. Focus on practical skills, such as operating AI tools and analyzing data. Upskilling your workforce ensures they feel confident and capable of leveraging AI effectively.
What are digital twins, and how do they benefit manufacturing?
Digital twins are virtual replicas of physical systems. They allow you to simulate and optimize processes before implementation. This technology reduces downtime, improves efficiency, and lowers costs. For example, you can test production changes in a digital environment without disrupting operations.
Previous Blogs
OutSystems AI Agent Tools Simplify Development Workflows
OutSystems AI Agent tools boost developer productivity by automating tasks, improving code quality, and cutting development time by up to 50%.
How to Master AI Agent Development on Solana
Discover how AI agents are revolutionizing blockchain on Solana, offering unmatched speed, scalability, and real-time solutions for decentralized finance and beyond.