ToB AI Agent Challenges and Practical Solutions
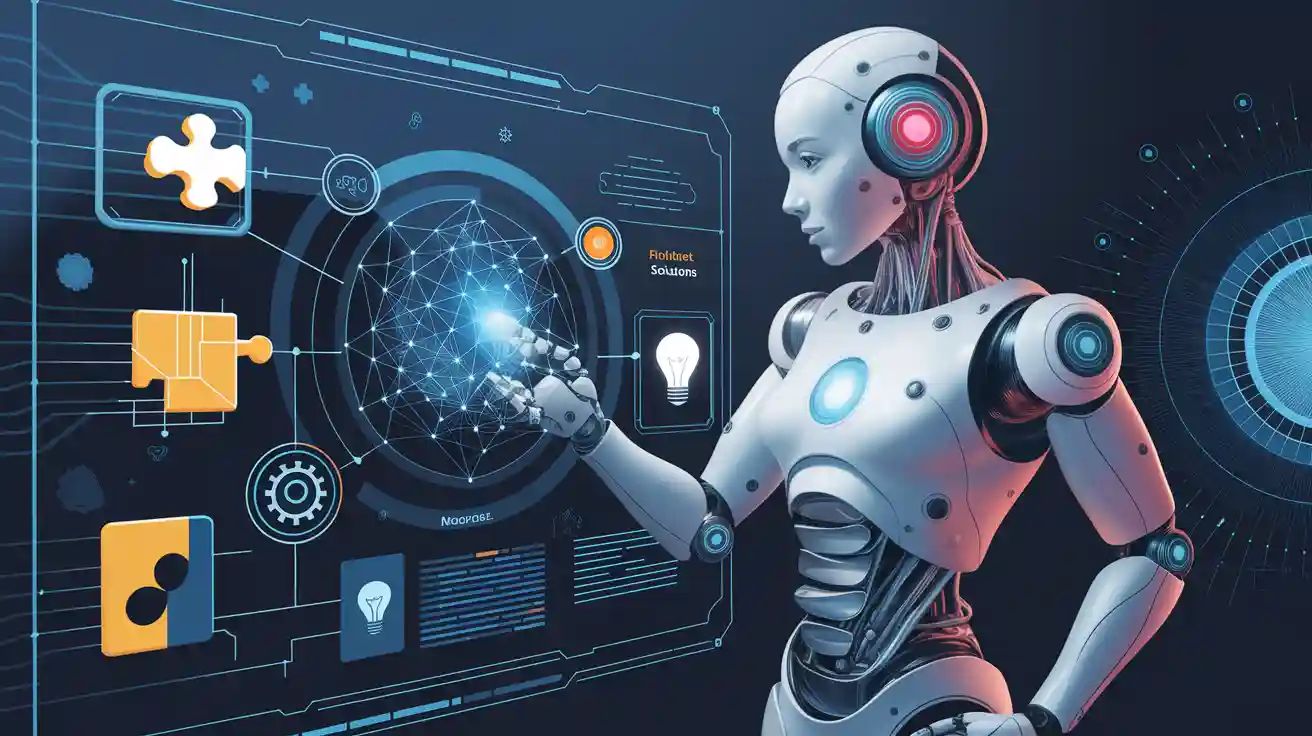
ToB AI agents transform modern business operations by automating complex workflows and enhancing productivity. Organizations increasingly recognize their potential, with 90% reporting improved workflows and employees experiencing a 61% efficiency boost. By 2030, these agents are expected to manage 80% of customer interactions, showcasing their growing importance. However, their implementation demands addressing critical challenges, including trustworthiness and adaptability. Solving these issues ensures reliable integration into diverse industries, unlocking their full potential for scalable and sustainable solutions.
Key Takeaways
- ToB AI agents help by automating tasks, making work faster. 90% of companies say their work got better.
- Fix tech problems by focusing on good data and using simple designs that fit with current systems.
- Use fair AI rules to gain trust, like checking systems often and being clear about choices.
- Use flexible tools like cloud platforms to save money and get the most from AI.
- Learn about new tech and rules to follow laws and improve AI use.
Key Challenges in ToB AI Agent Applications
Technical Challenges
ToB AI agents rely on advanced algorithms and large-scale models, but technical hurdles often impede their effectiveness. One major challenge is integrating these agents with legacy systems. Many enterprises operate on outdated infrastructure, which lacks compatibility with modern AI technologies. This mismatch can lead to operational disruptions and increased costs during implementation.
Another technical issue involves data quality and availability. Poor-quality or insufficient data can hinder the training and performance of AI agents. For example, incomplete datasets may result in inaccurate predictions or unreliable decision-making processes. Additionally, algorithmic bias poses a significant risk. AI systems can inadvertently learn and perpetuate biases present in training data, leading to unfair outcomes and ethical concerns.
Tip: Enterprises should prioritize data governance and invest in modular AI designs to ensure seamless integration and adaptability.Operational Challenges
Operational challenges arise when deploying ToB AI agents in dynamic business environments. One common issue is scalability. While AI agents excel in handling specific tasks, scaling their capabilities across diverse workflows often requires extensive customization. This process can be time-consuming and resource-intensive.
Another challenge involves workforce adaptation. Employees may resist adopting AI-driven workflows due to concerns about job displacement or lack of familiarity with the technology. Training programs and clear communication are essential to address these concerns and foster collaboration between human workers and AI agents.
Moreover, maintaining the reliability of AI agents in real-time operations can be difficult. Unexpected scenarios or edge cases may cause the agents to fail, requiring human intervention to resolve issues.
Ethical and Compliance Issues
Ethical considerations play a crucial role in the widespread adoption of ToB AI agents. Algorithmic bias, as mentioned earlier, can lead to discriminatory practices, especially in sensitive areas like hiring or financial services. Ensuring fairness and transparency in AI decision-making is vital to building trust among stakeholders.
Compliance with data privacy regulations adds another layer of complexity. AI agents often process vast amounts of sensitive information, making them susceptible to security breaches. Enterprises must implement robust cybersecurity measures and adhere to legal frameworks like GDPR or CCPA to protect user data.
Note: Ethical AI practices, including regular audits and transparent reporting, can help organizations mitigate risks and align with compliance standards.Challenge | Description |
---|---|
Data Quality and Availability | Poor-quality or insufficient data can lead to ineffective AI agents, resulting in incorrect answers and poor user experiences. |
Algorithmic Bias | AI can learn and perpetuate biases present in training data, leading to ethical concerns and unfair outcomes. |
Integration with Existing Systems | Technical roadblocks can arise when integrating AI agents with legacy systems, causing operational disruptions. |
Cost and Resource Constraints
Implementing ToB AI agents often requires significant financial and resource investments, which can pose challenges for businesses. The development and deployment of these agents demand advanced infrastructure, skilled personnel, and ongoing maintenance. For small and medium-sized enterprises (SMEs), these costs can become a barrier to adoption.
One major expense involves the initial setup. Businesses must invest in robust hardware and software systems to support AI operations. Additionally, training AI agents on industry-specific data requires time and resources. Companies may also need to hire or upskill employees to manage and optimize these systems effectively.
Another challenge lies in the operational costs. AI agents consume substantial computational power, especially when processing large datasets or performing complex tasks. This can lead to increased energy consumption and higher operational expenses. Furthermore, scaling AI solutions across multiple departments or locations often requires additional investments in infrastructure and integration efforts.
To address these constraints, businesses can adopt cost-optimization strategies. For instance, leveraging cloud-based AI platforms can reduce the need for expensive on-premises infrastructure. Modular AI designs also allow companies to implement solutions incrementally, spreading costs over time. Collaborating with third-party providers for specialized AI services can further alleviate financial burdens.
Tip: Prioritizing scalable and flexible AI solutions enables businesses to manage costs effectively while maximizing the benefits of ToB AI agents.Data Privacy and Security Concerns
Data privacy and security represent critical challenges in the deployment of ToB AI agents. These agents, built on large language models (LLMs), face numerous security threats, including classic software vulnerabilities. Their ability to interact with external systems increases the risk of data breaches and privacy violations.
AI agents often process sensitive information, such as customer data, financial records, and proprietary business details. Without robust security measures, this data becomes vulnerable to unauthorized access or cyberattacks. Common risks include prompt injection, sensitive data leakage, and supply chain vulnerabilities. Additionally, integrating external tools exposes systems to threats like SQL injection, remote code execution, and broken access control.
Concern Type | Percentage of Respondents |
---|---|
Trust in AI agents | 85% |
Data security and privacy | Most cited barrier |
To mitigate these risks, organizations must implement comprehensive cybersecurity frameworks. Encrypting data during transmission and storage ensures its protection from unauthorized access. Multi-factor authentication and regular security audits further enhance system integrity. Adhering to data privacy regulations, such as GDPR or CCPA, also helps businesses maintain compliance and build trust with stakeholders.
Note: Regularly updating AI systems and conducting vulnerability assessments can prevent potential security breaches and ensure the safe operation of ToB AI agents.Practical Solutions to Overcome Challenges
Addressing Technical Challenges with Modular Design
Modular design offers a practical approach to overcoming technical challenges in ToB AI agent deployment. By breaking down complex systems into smaller, independent modules, businesses can enhance flexibility and scalability. Each module can focus on a specific function, such as data processing, decision-making, or tool integration. This approach simplifies troubleshooting and allows for incremental updates without disrupting the entire system.
For example, modular AI designs enable organizations to adapt to changing business needs. If a company requires additional functionality, it can integrate a new module without overhauling the existing infrastructure. This reduces downtime and minimizes costs. Furthermore, modular systems support collaboration between different teams, as each module can be developed and maintained independently.
Tip: Businesses should prioritize modular architectures to ensure seamless upgrades and compatibility with future technologies.Streamlining Integration with Legacy Systems
Integrating ToB AI agents with legacy systems remains a significant challenge for many organizations. However, adopting strategic methods can streamline this process and ensure smooth operations. Middleware solutions, for instance, act as a bridge between modern AI technologies and outdated infrastructure. These solutions enable data exchange and functionality without requiring a complete system overhaul.
Several companies have successfully implemented AI agents alongside legacy systems. For instance:
Company | Integration Method | Outcome |
---|---|---|
Global Insurance Co. | Used middleware to modernize a COBOL-based claims system | Overcame limitations of legacy system |
American Express | Implemented AI for real-time transaction analysis | Enhanced security and transaction processing |
US Consumer Bank | Connected AI with legacy systems | 50% drop in card fraud, 99.95% system availability |
These examples highlight the importance of tailored integration strategies. Middleware not only ensures compatibility but also enhances system performance. Additionally, businesses can leverage APIs to connect AI agents with existing software, enabling real-time data processing and decision-making.
Note: Regular testing and monitoring during integration can prevent disruptions and ensure optimal performance.Ensuring Ethical AI Practices and Governance
Ethical AI practices are essential for building trust and ensuring the reliability of ToB AI agents. Organizations must establish robust governance frameworks to address issues such as algorithmic bias, transparency, and accountability. These frameworks should include guidelines for ethical decision-making, regular audits, and tools for bias detection.
Leading companies have implemented governance frameworks to promote ethical AI practices:
Organization | Governance Framework | Key Features |
---|---|---|
Microsoft | AETHER Committee | Multidisciplinary review, Responsible AI Standard, Fairness Checklist |
IBM | AI Ethics Board | Compliance with responsible AI principles, AI fairness tools |
AI Principles | Internal controls for high-risk AI, What-If Tool for bias analysis |
These frameworks demonstrate the importance of proactive measures in managing AI systems. For instance, Microsoft's AETHER Committee conducts multidisciplinary reviews to ensure fairness and compliance. Similarly, IBM's AI Ethics Board uses fairness tools to identify and mitigate biases in AI models.
Tip: Organizations should adopt similar governance frameworks to align their AI practices with ethical standards and regulatory requirements.Optimizing Costs Through Scalable Solutions
Cost optimization is a critical factor for businesses deploying ToB AI agents. Scalable solutions allow organizations to manage expenses effectively while maximizing the benefits of AI integration. By adopting strategic approaches, companies can reduce upfront investments and operational costs without compromising performance.
One effective strategy involves leveraging pre-trained AI agents. These models eliminate the need for building systems from scratch, significantly reducing development expenses. Additionally, businesses can optimize data collection by utilizing synthetic data or existing datasets, which minimizes the costs associated with data labeling and preparation.
Another cost-saving measure is the use of edge AI. Deploying AI on local devices reduces reliance on cloud infrastructure, cutting down on storage and processing expenses. Similarly, transfer learning enables organizations to build on existing models, saving time and resources during training. Progressive development, starting with a Minimum Viable AI Agent, further prevents over-investment by allowing incremental improvements based on real-world feedback.
The following table outlines scalable solutions that support cost optimization:
Strategy | Description |
---|---|
Use Pre-Trained AI Agents | Leverage existing models to reduce development costs. |
Optimize Data Collection | Use synthetic data and existing datasets to lower labeling costs. |
Reduce API Call Costs | Optimize API usage to minimize operational expenses. |
Use Edge AI | Deploy AI on local devices to cut cloud costs. |
Implement Transfer Learning | Build on existing models to save on training costs. |
Optimize Infrastructure | Use cost-effective hosting solutions and auto-scaling. |
Focus on Progressive Development | Start with a Minimum Viable AI Agent to avoid over-investment. |
Use Simulations for Training | Train in simulated environments to reduce real-world costs. |
Automate Monitoring | Use tools to automate model maintenance and reduce manual costs. |
Strengthening Data Privacy and Cybersecurity Measures
Data privacy and security are paramount when deploying ToB AI agents. These systems often handle sensitive information, such as customer data and proprietary business details, making them prime targets for cyberattacks. Organizations must implement robust measures to safeguard data and maintain trust.
AI-driven prompt security is one effective measure. It blocks inappropriate queries and prevents the disclosure of sensitive information. Real-time content filtering ensures that user inputs and AI outputs remain appropriate, reducing the risk of data leaks. Federated learning techniques further enhance model performance while preserving data privacy by keeping sensitive information decentralized.
The table below highlights key security measures for protecting ToB AI agents:
Security Measure | Description |
---|---|
AI-driven prompt security | Blocks inappropriate queries and prevents sensitive information disclosure. |
Real-time content filtering | Ensures appropriateness of user inputs and AI outputs. |
Federated learning techniques | Enhances model performance while maintaining data privacy. |
Organizations should also adopt comprehensive cybersecurity frameworks. Encrypting data during transmission and storage protects it from unauthorized access. Multi-factor authentication adds an extra layer of security, while regular vulnerability assessments help identify and address potential risks. Adhering to data privacy regulations, such as GDPR or CCPA, ensures compliance and builds stakeholder confidence.
Note: Trust in AI agents' ability to handle critical enterprise data securely is essential for their widespread adoption. Regular updates and audits can further enhance system integrity.Case Studies and Examples
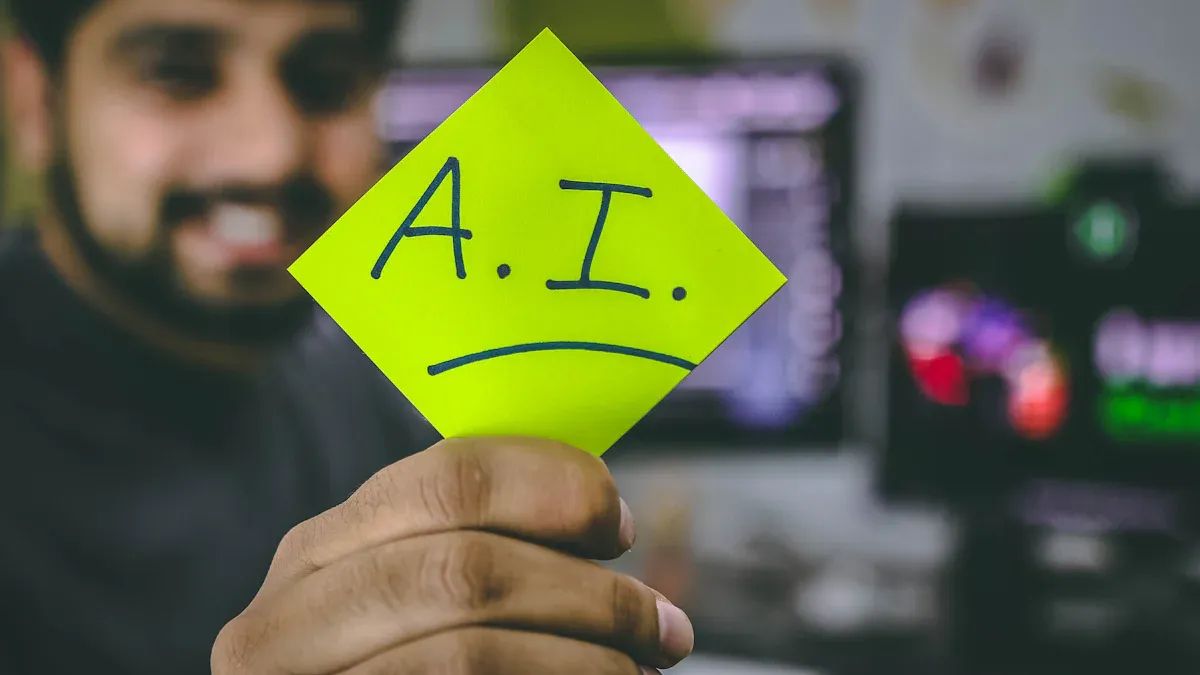
AI Agent Deployment in Supply Chain Optimization
AI agents have revolutionized supply chain management by enhancing efficiency and accuracy. Companies like Amazon utilize AI-driven systems to analyze vast datasets in real time, enabling precise demand forecasting. These systems predict demand shifts with up to 96% accuracy, allowing businesses to adjust inventory levels proactively.
Key performance indicators highlight significant improvements. Inventory optimization precision has reached 94%, reducing excess stock and associated costs. Logistics expenses have decreased by 45%, while delivery delays have dropped by 42%. These advancements demonstrate the transformative impact of AI agents on supply chain operations.
Tip: Businesses can leverage AI agents to streamline supply chain workflows, reduce costs, and improve customer satisfaction.Enhancing Customer Support with AI Agents in B2B Services
AI agents play a pivotal role in improving customer support for B2B services. Companies integrating AI into Voice of Customer (VoC) programs report a 30% improvement in responding to feedback. Coca-Cola, for instance, uses AI-driven VoC systems to analyze customer input across multiple channels. This approach enables swift identification and resolution of issues, enhancing customer experience.
Overall satisfaction levels increase by 25% when AI agents are deployed. These systems provide personalized responses and faster resolutions, fostering stronger client relationships. By automating repetitive tasks, AI agents free human representatives to focus on complex inquiries, ensuring a balanced and efficient support system.
Note: AI agents empower businesses to deliver exceptional customer service while optimizing operational efficiency.Automating Financial Processes with AI Agents
AI agents streamline financial operations by automating complex workflows. Leading organizations like Revolut and Mastercard employ AI for tasks such as KYC processes and real-time transaction monitoring. Revolut's facial recognition technology expedites onboarding, while Mastercard's machine learning models detect suspicious transactions instantly, preventing fraud.
Application Area | Key Benefits |
---|---|
Wealth Management | Tailored investment advice enhances compliance and optimizes strategies. |
Regulatory Reporting Automation | Intelligent solutions minimize errors and ensure timely submissions. |
Payment Processing Optimization | Streamlined workflows reduce transaction times and errors. |
These applications improve operational efficiency, reduce costs, and enhance customer satisfaction. AI agents also excel in risk assessment, providing accurate insights for credit and investment decisions. Their ability to automate compliance processes ensures adherence to regulations while minimizing manual effort.
Tip: Financial institutions can adopt AI agents to boost productivity, reduce risks, and deliver personalized services.Future Trends and Recommendations
Emerging Technologies in ToB AI Agent Development
The development of ToB AI agents is evolving rapidly, driven by emerging technologies and market trends. Big tech companies are leading the charge by enhancing general-purpose agents, making them more accessible and cost-effective for businesses. Simultaneously, the private AI agent market is witnessing increased specialization. Companies are focusing on niche areas such as customer support and coding, resulting in tailored, industry-specific solutions.
The structuring of AI agent infrastructure is another significant trend. Developers are creating specialized solutions for various aspects of AI agent development, such as memory, planning, and tool integration. This structured approach simplifies deployment and enhances reliability. Furthermore, organizations are shifting from experimentation to implementation. Despite challenges in reliability and security, many businesses now prioritize deploying AI agents to achieve tangible results.
Trend Description | Key Insights |
---|---|
Big tech dominance in general-purpose agents | Enhanced accessibility and cost-effectiveness. |
Specialization in private AI agent market | Focus on niche areas like customer support and coding. |
Structuring of AI agent infrastructure | Organized solutions for development aspects like memory and planning. |
Shift from experimentation to implementation | Increased focus on practical deployment despite challenges. |
Preparing for AI Regulation and Compliance
As AI adoption grows, regulatory frameworks are becoming more stringent. Governments and organizations are introducing policies to ensure ethical AI practices, data privacy, and accountability. Businesses must prepare for these changes by implementing proactive compliance strategies.
One effective approach involves conducting regular audits to identify potential risks and ensure adherence to regulations like GDPR and CCPA. Establishing clear governance frameworks can also help organizations manage ethical concerns, such as algorithmic bias and transparency. Additionally, businesses should invest in training programs to educate employees about compliance requirements and ethical AI practices.
Note: Staying ahead of regulatory changes not only ensures compliance but also builds trust with stakeholders.Building a Scalable and Sustainable AI Strategy
Developing a scalable and sustainable AI strategy requires a structured approach. Frameworks like the OODA Loop emphasize agility and iterative improvement, enabling businesses to adapt quickly to changing needs. The AI Maturity Model provides a stepwise guide for assessing readiness, from initiation to optimization.
Promoting a data-driven culture is another critical factor. Organizations should encourage informed decision-making by integrating AI into everyday workflows. Pilot projects offer a practical way to test small-scale AI initiatives before full implementation, reducing risks and ensuring alignment with business goals.
Framework | Description |
---|---|
OODA Loop | Focuses on agility and iterative improvement. |
AI Maturity Model | Assesses readiness for AI transformation in stages. |
Assessment and Alignment | Evaluates current processes to identify AI value addition. |
Data Culture Adoption | Encourages informed decision-making through data-driven practices. |
Pilot Projects | Tests small-scale AI initiatives before full implementation. |
ToB AI Agent applications face challenges like data quality, ethical concerns, and integration hurdles. Solutions such as modular design, scalable strategies, and robust governance frameworks address these issues effectively. Ethical practices and secure systems ensure trustworthiness, while scalable solutions optimize costs.
Businesses must stay informed about emerging technologies and regulations. Proactive adoption of AI agents fosters innovation, enhances efficiency, and ensures sustainable growth in competitive markets.FAQ
What are ToB AI agents, and how do they differ from traditional AI tools?
ToB AI agents are autonomous systems designed for business applications. Unlike traditional AI tools, they integrate planning, memory, and tool usage to execute tasks independently. They act as "digital employees," enhancing workflows and decision-making processes across industries.
How can businesses ensure the ethical use of ToB AI agents?
Organizations should implement governance frameworks that include bias detection tools, regular audits, and transparent reporting. Ethical AI practices ensure fairness, accountability, and compliance with regulations like GDPR. Proactive measures build trust among stakeholders and mitigate risks.
What industries benefit most from ToB AI agents?
Industries like finance, supply chain management, and customer support gain significant advantages. AI agents optimize workflows, reduce costs, and enhance decision-making. For example, they streamline financial processes, improve inventory management, and provide personalized customer service.
Are ToB AI agents secure for handling sensitive data?
Yes, with proper measures. Businesses should use encryption, multi-factor authentication, and regular vulnerability assessments. Federated learning and real-time content filtering further enhance security. Adhering to data privacy regulations ensures safe operations and builds trust.
How can companies manage the costs of deploying ToB AI agents?
Cost optimization strategies include leveraging pre-trained models, using edge AI, and implementing transfer learning. Modular designs allow incremental deployment, reducing upfront investments. Cloud-based platforms and progressive development further minimize expenses while maximizing efficiency.
Previous Blogs
How to Perform RL Scaling Using RAG for Knowledge Enhancement
Learn how to combine RAG and RL scaling to optimize AI systems, improve knowledge retrieval, and enhance performance across diverse tasks and industries.
What is Edge AI Computing and How It Works
Edge AI computing integrates AI with edge devices to process data locally, enabling real-time decisions, reducing latency, and enhancing privacy.